Data Fabric: Heart of Data centric Enterprise
Over the past decade, we have been hearing a lot of terminologies like Cloud Enterprise Data Warehouse(Cloud EDW), Data Lakes, Data Mesh, Data Lakehouses, and Data Fabric. Let’s get these terminologies out of the way, as there are tons and tons of them out there, and all can sound the same. It’s better to categorize them into methodologies and tools.
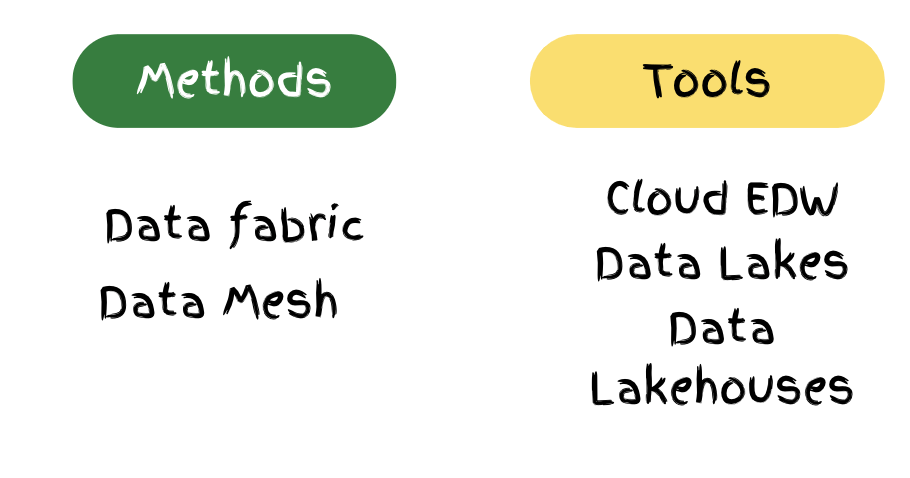
If we look at the tool’s side, we have a cloud data warehouse or enterprise data warehouse. These are large central repositories for clean and structured business analytical data. Then we have data lakes, that have gathered attraction in the past decade or so, due to expansion in unstructured data sources. Data Lakes are a great source to dump all the raw data quickly and then use it for analysis later.
Next and last in the tools example section is Data Lakehouse which has emerged over the past few years. This carries the flexibility and cost-efficiency of data lakes with the data management capability of a data warehouse thereby enabling BI and ML on all of the data. So all these mentioned on the tool side have great capability of analytical and operational reporting but it comes to the cost of moving or copying data to a central repository. This creates challenges with data governance, data quality and, most importantly proliferate data silos. Due to these challenges, a broader data strategy has become a need of an hour for a Data-Centric Enterprise.
What is Data Fabric?
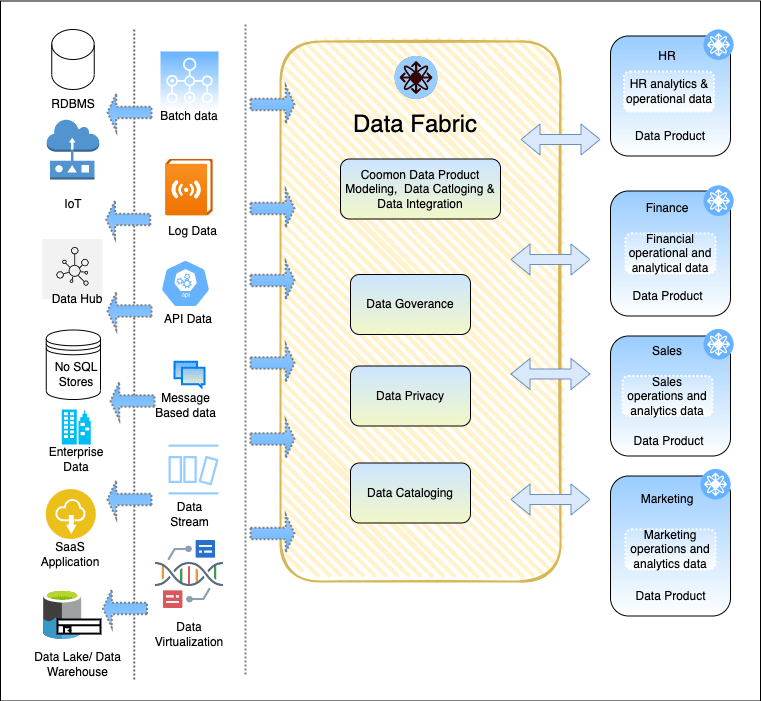
It’s an architectural approach and set of technologies that allow you to break down data silos and get data into the hands of data users. It enables accessing, ingesting, integrating, and sharing data across an enterprise in a governed manner regardless of location whether it’s an on-premises system or multiple cloud environments.
Three major responsibilities or roadmap items of data fabric :
- Accessing Data: The first responsibility of the data fabric is to provide access to different data sources present at a different locations. Data can be present in data Lakes, EDW, RDMS, or SAAS applications. A data fabric allows you to have aggregate access to all these data sources through a virtualization layer without moving or copying tons of data from one location to another.
- Robust Data Integration Tool: Sometimes there is a need to copy data. Perhaps the application you are building has a certain latency requirement or requires a formal data pipeline. In that scenario, Data Fabric must have robust ETL tools and data integration tools. We can clean and transform the data and get it to the central repository where user can have aggregate access with other data sources
- Managing Life Cycle of our data: This has two parts, governance, and compliance.
- Governance: For governance, we need to make sure that the right folks have the right access to data and nothing more. Data fabric uses active metadata to automate policy enforcement. The policies can be masking certain aspects of the dataset or some information that we want to redact. These policies can be defined based on role-based access control.
- Rich data Lineage: Data Fabric should provide rich data lineage as in from where the data comes from, and what transformation was done on the data and then we can start using it for data quality and assurance purposes.
- Compliance: There is a lot of data compliance coming to attention with the growth of a data-centric organization. There are compliance like GDPR, CCPA, HIPPA (if the health care industry) , and FCRA (Financial Services). Data Fabric helps in defining these compliance policies.
- Exposing Data: After the data sources are connected and our governance policies are well-defined, we would like to expose the data to the end users.
- Enterprise Data Catalog: The data should flow through multiple enterprise catalogs and reach the end users. The end user can be a data scientist, Business Analyst, Application developer, BI Analyst, Or Predictive/ML Engineer.
- Multiple Support: The data fabric should support different vendors for this platform and also support open source technologies and also support Application developers to build custom applications by exposing data from the catalog through different API
There are also concepts of data mesh and data virtualization. People often confuse Data Virtualization with Data fabric and treat data mesh similar to Data Fabric. Data Virtualization is one of the technologies that enables Data fabric. Data mesh focuses on organizational frameworks while data fabric is a technology-centric solution
Data Fabric Vs Data Virtualisation
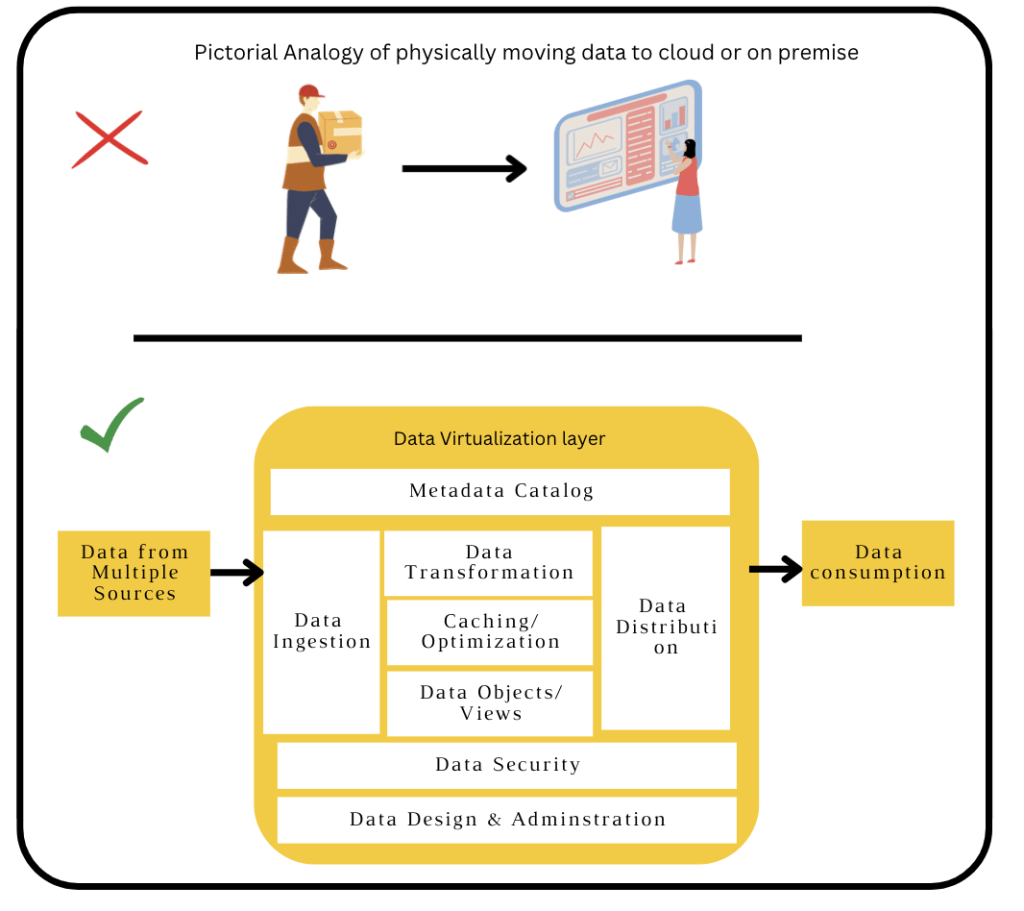
Data fabric mostly gets confused with Data Virtualization. So let’s understand Data virtualization and how it enables Data Fabric Approach.
- Data Virtualization has changed the way data gets to the hands of analysts, data scientists, businesses, or applications.
- Rather than physically moving the data to the cloud or on-premise, it creates an abstraction layer or to say a Data Virtualization layer.
- So it connects to different data sources, ingests data, performs ETL processes, and creates a virtual data layer and thereby allowing users to leverage the data in real-time from multiple sources.
Now you must be thinking that similar things we do in Data Fabric. We don’t do similar things in Data Fabric, but to say we do more in Data Fabric. So, Data Virtualization is just one of the technology that enables Data Fabric.
Data Fabric is an overarching end-to-end data management architecture where the goal or the use case is not just getting data on the cloud or in the hand of business analysts but dealing with broader use cases like customer intelligence or customer 360-degree view or IoT analytics. Data Fabric works on a larger stack of technologies.
Data Fabric Vs Data Mesh
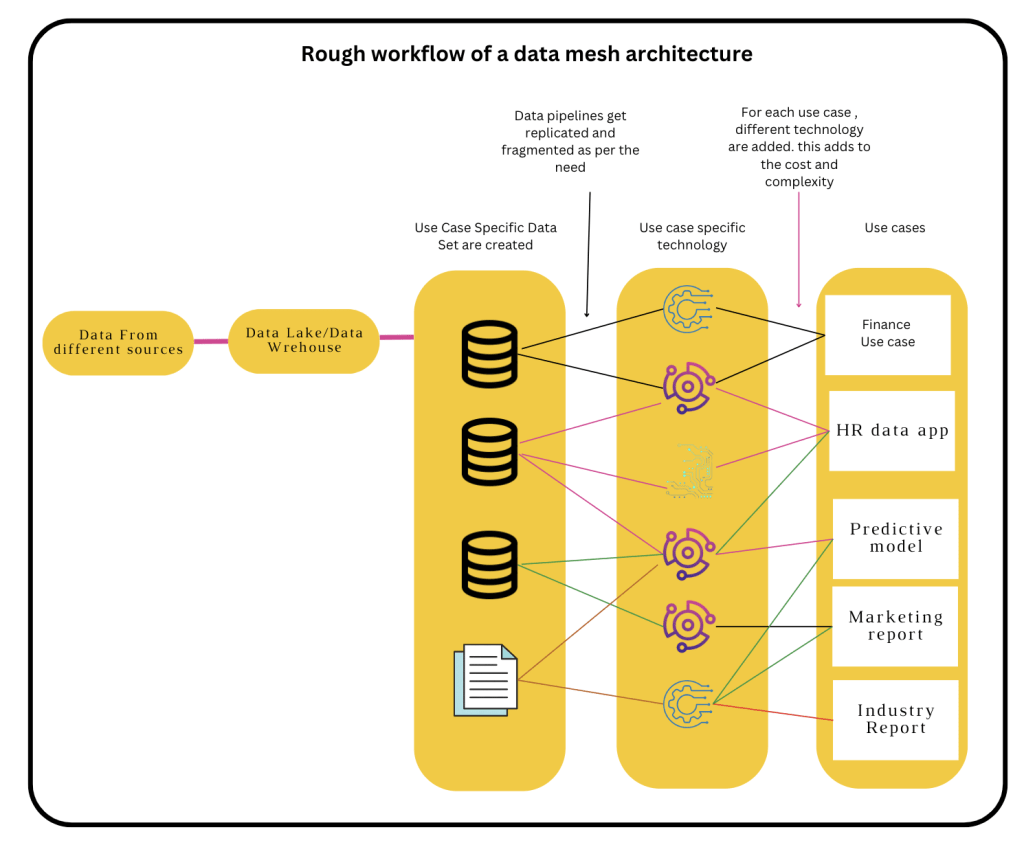
According to Forrester, “A data mesh is a decentralized sociotechnical approach to share, access, and manage analytical data in complex and large-scale environments—within or across organizations using.” [1]
The concept of data mesh is a framework that focuses on data ownership that is appropriate for SMEs owning and managing the data end to end (Top Down Data management approach).
With every other business, you see data ownership is decentralized and various business domains or functions would like to create their data product. These data products and API are then interchangeably used by data scientists and other data consumers through API. The Data Mesh Architecture approach creates an alignment of data sources from various business domains and functional units to their data owners.
The best value of the Data Mesh approach I see is that it shifts the creation of the data product from data engineers to the hands of Data owners. There is a potential flaw in that approach if data mesh is not managed properly then it can accelerate data silos. So for successful data mesh, there must be a supported organizational structure among the aligned SMEs.
Action Plan for Data Fabric Implementation to Generate Business Value
Often the discussion about big data, cloud technologies , Data Fabric are tightly interrelated. What we see missing is a related integrated conversation on change in data owners mindset or management practices. Those are the conversation, that transition Data fabric to not just a technical implementation but the one that generates business value.
Here are few steps that to go beyond implementation to value
- Invest on Data Fabric as a Product and not a project: A technology implementation as a project will not work for Data Fabric. Once a technical implementation of Data Fabric is achieved without any measurable business outcome then thats not a victor, rather treating it as a product and even first iteration of it results in some business value then thats a victory.
- Focus on Agile Implementation : Agile implementation will help in coping up with the complexity of its implementation & execution. So build a little, test a little, deploy a little, launch a little.
- Data Fabric as a Service: If we run data fabric as a service to consumer request, then this will help us not employ data fabric experts. It should be a service, which consumers of the data uses to get the results they asked for.
Add Comment
You must be logged in to post a comment.
Nishkarsh Raj
Insightful writeup. Thanks for sharing 🔥
Nishkarsh Raj
Insightful writeup. Thanks for sharing 🔥