RecommendoSphere: Pioneering User-Centric Suggestions
Introduction
Personalized content recommendations are provided by recommender systems, which are crucial in modern applications and have a significant impact on user experiences across several industries. In a nutshell, recommender systems help you discover new things you’ll like by either comparing your preferences to others (collaborative filtering) or by finding similar items based on what you’ve liked before (content-based filtering). They make your online experience more personalized and enjoyable!
In this blog, recommender systems are thoroughly investigated to understand their multidimensional relevance and crucial function in influencing user preferences.
The growing impact of recommender systems on decision-making procedures and user involvement serves as the driving force behind this study. Understanding these systems’ underlying workings is crucial as they continue to influence our digital interactions. The rationale for conducting this research is the desire to understand the complex algorithms that underlie suggestions.
The blog outlines a distinct scope for this investigation and states its specific goals. The scope includes a thorough examination of the approaches used by recommender systems, their development over time, and their applications in diverse fields. This thesis seeks to offer a comprehensive knowledge of the difficulties and possibilities present in recommender systems, offering insightful contributions to the larger field of machine learning applications.
The goals are to analyze various recommender system types, assess their performance measures, and investigate the ethical issues and difficulties they provide. This blog aims to provide readers with a thorough grasp of recommender systems’ consequences in contemporary technological landscapes by defining a clearly defined scope and objectives.
Enabling Personalization
- Recommender systems actively work to provide users with tailored recommendations. Their primary goal is to present users with content, products, or services that align closely with their individual preferences and needs. This personalization not only enhances user satisfaction but also boosts engagement.
- Recommender systems aim to provide personalized recommendations. For instance, think about a music streaming service like Spotify. It analyzes your listening history and preferences to suggest songs and playlists that match your taste. When you open the app, you’re greeted with playlists like “Discover Weekly” or “Your Daily Mix,” tailored specifically to your music preferences.
Enhancing User Experiences
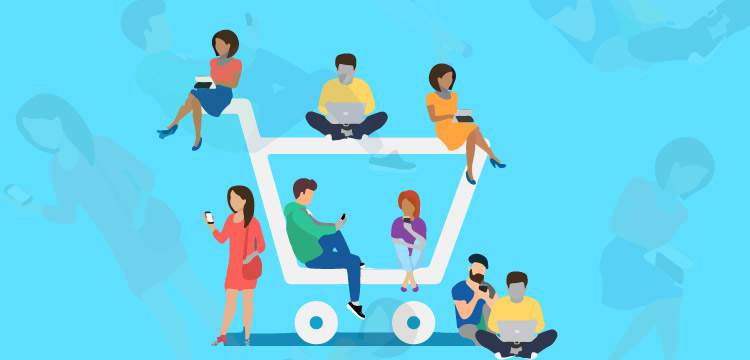
- Recommender systems contribute significantly to improving the overall user experience. They enable users to quickly discover desired items and uncover new points of interest. By offering tailored suggestions that resonate with users’ tastes and preferences, these systems create a more enjoyable and efficient user journey.
- Consider an e-commerce website like Amazon. When you shop online, Amazon recommends products based on your previous purchases and browsing history. These recommendations help you quickly find items you’re interested in, creating a smoother and more enjoyable shopping experience.
Boosting User Engagement
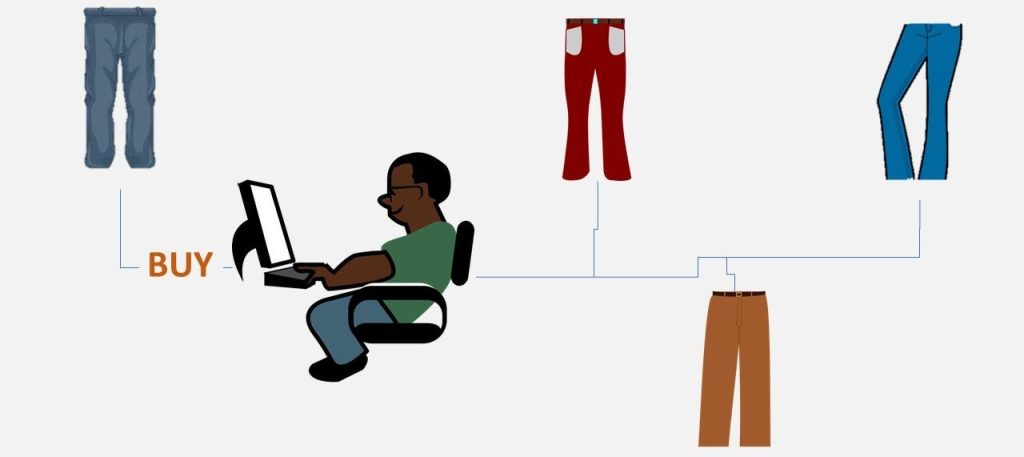
- Personalized recommendations have the remarkable ability to increase user engagement. When users encounter content or products that align with their interests, they are more inclined to spend more time on a website or platform. They explore additional items and make repeat visits, strengthening their engagement.
- Social media platforms like Facebook and Instagram use recommender systems to keep users engaged. They analyze your interactions, such as the posts you like and the people you follow, to show you content from friends and pages that are most relevant to you. This keeps you scrolling, liking, and commenting, leading to increased user engagement.
Facilitating Customer Retention
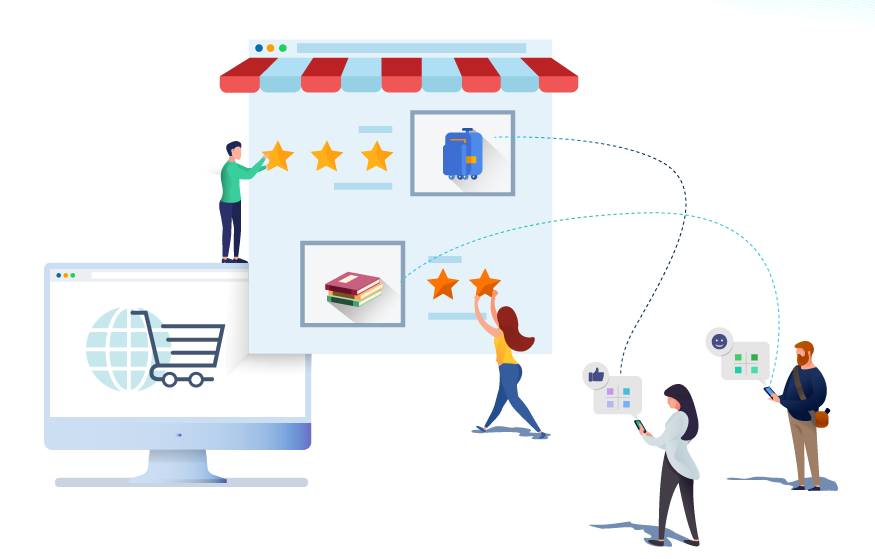
- Recommender systems are pivotal in retaining users and customers. By consistently delivering valuable and personalized recommendations, businesses can nurture user loyalty. This reduces churn rates, ensuring that users continue to engage with the platform and maintain enduring relationships.
- Streaming services like Netflix excel at retaining customers through recommender systems. By suggesting movies and TV shows tailored to your viewing history and preferences, they encourage you to continue your subscription and stay loyal to their platform.
Enhancing Conversion Rates
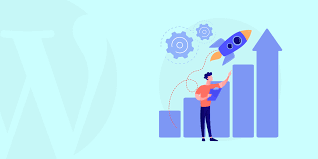
- In e-commerce and retail, recommender systems wield significant influence over conversion rates. When users receive product recommendations that align with their preferences, they are more likely to make purchases. This results in increased sales and revenue for businesses.
- In e-commerce, recommender systems can significantly impact conversion rates. For instance, if you’re shopping in an online clothing store, the system may recommend accessories or shoes that complement the clothing items you’re browsing. This increases the likelihood of you making additional purchases and boosts the store’s sales.
Promoting Content Discovery
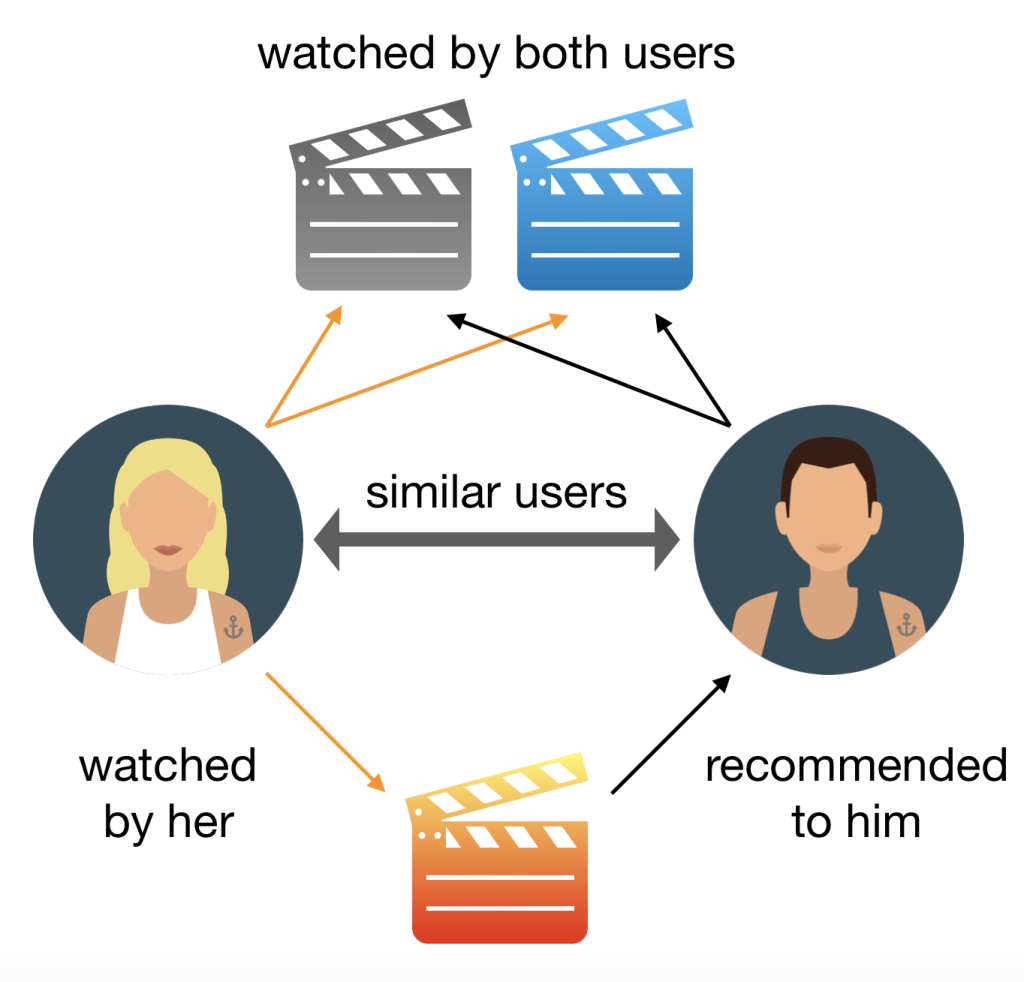
- Recommender systems actively assist users in discovering a diverse range of content. Whether it’s articles, videos, music, or books, these systems guide users toward relevant and interesting content they might not have discovered on their own.
- YouTube is a prime example of a platform that promotes content discovery. Its recommender system suggests videos related to the ones you’ve watched, introducing you to new content creators and topics you might not have found otherwise.
Empowering Cross-Selling and Upselling
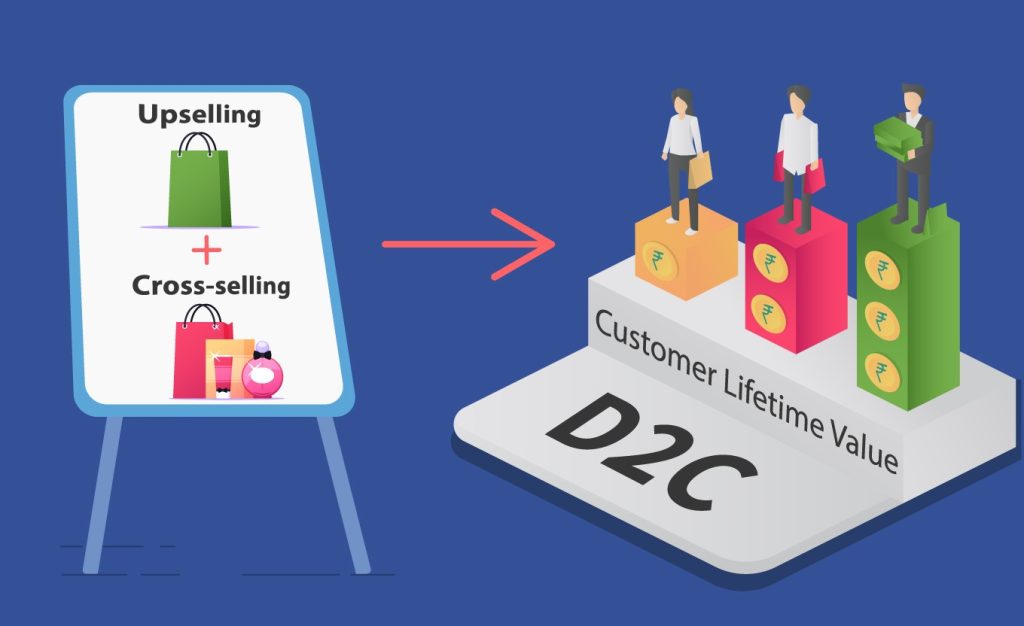
- Recommender systems play a strategic role in suggesting related or complementary products or services. This empowers businesses to engage in cross-selling and upselling, increasing the average transaction value.
- Online marketplaces like Amazon use cross-selling and upselling techniques. When you view a product, the system suggests complementary items (cross-selling) or higher-end versions of the product (upselling). For instance, if you’re looking at a camera, you may recommend a camera bag and a better lens.
Mitigating Information Overload
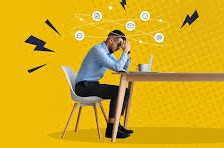
- In today’s information-rich world, users often face an overwhelming number of choices. Recommender systems effectively sift through and organize these options, simplifying the user’s quest for desired content.
- News websites like The New York Times employ recommender systems to help readers navigate the vast amount of content available. They recommend articles based on your interests and reading history, making it easier for you to find relevant news amidst the multitude of articles.
Boosting Business Efficiency
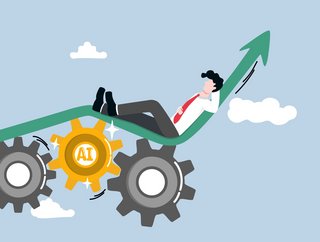
- Recommender systems streamline the process of suggesting items to users, reducing the need for manual curation and recommendation. This automation saves time and resources for businesses, allowing them to operate more efficiently.
- Email marketing platforms like MailChimp use recommender systems to automate personalized email campaigns. They analyze subscriber behavior and preferences to send targeted promotions, saving time and resources for businesses while increasing the effectiveness of their marketing efforts.
Gaining Competitive Advantage
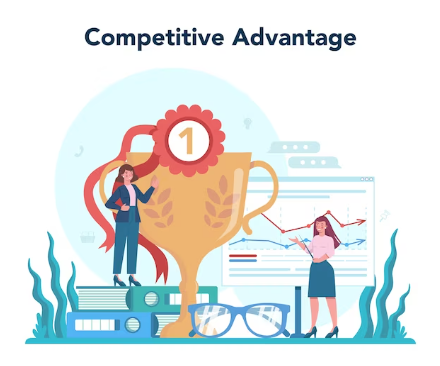
- Organizations that skillfully implement recommender systems gain a competitive edge. They can offer superior recommendations compared to competitors, attracting and retaining a larger user or customer base.
- Streaming platforms like Netflix and Amazon Prime Video compete by offering better recommendations. Netflix’s recommendation system, for example, helps it stand out among competitors by providing users with highly personalized content suggestions.
Leveraging Data
- Recommender systems effectively utilize user data and feedback to optimize their recommendations. By analyzing user interactions and preferences, businesses gain valuable insights into user behavior. This information enables them to tailor their offerings and marketing strategies accordingly.
- Social networks like LinkedIn leverage user data and interactions to recommend relevant job openings and connections. By analyzing your profile and activities, LinkedIn suggests jobs that match your skills and interests, making it a valuable tool for job seekers.
Personalized Marketing
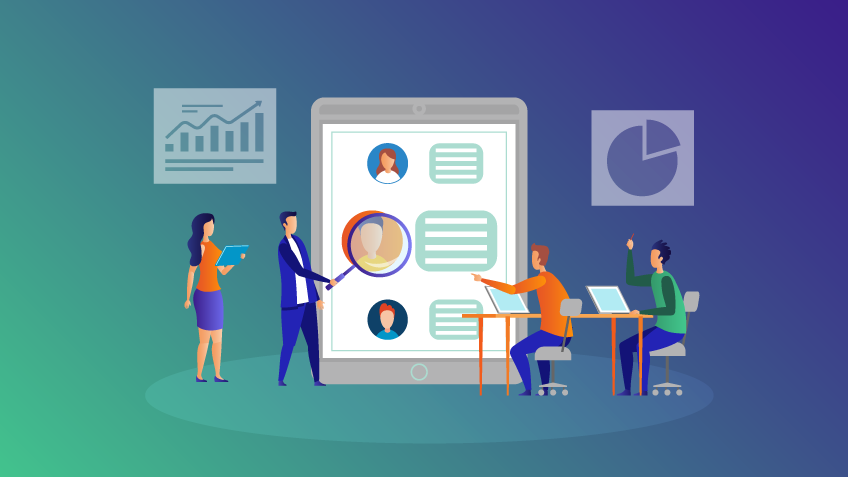
- Recommender systems are instrumental in personalized marketing efforts. Businesses can use them to send targeted promotions and advertisements to users based on their interests and past interactions. This increases the likelihood of conversion.
- Online retailers like Amazon use recommender systems for personalized marketing. They send you emails with product recommendations based on your past purchases and browsing history, increasing the chances of you making a purchase.
Recommender systems are versatile tools used across various industries to provide personalized recommendations, enhance user experiences, boost engagement, increase sales, and streamline processes. They leverage data and user behavior to optimize content and product suggestions, making them an integral part of many online platforms and businesses.
Recommender systems come in various types, each with its approach to making recommendations
Collaborative Filtering
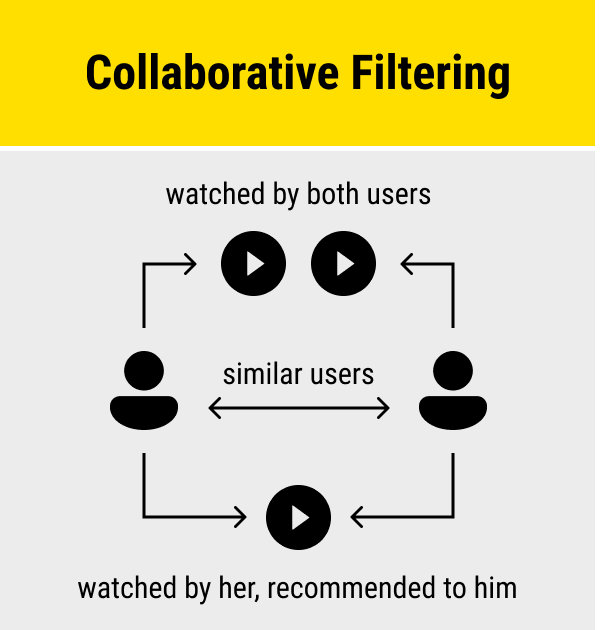
This type of recommender system suggests items based on the preferences and behaviors of similar users. It assumes that users who liked similar things in the past will continue to do so. For example, if two users have enjoyed similar movies in the past, collaborative filtering might suggest new movies to one user based on the other’s preferences.
Imagine you are using a movie streaming service like Netflix. Collaborative filtering would recommend movies to you based on what users with similar viewing histories and preferences have liked. For example, if you and another user both enjoyed action and adventure movies like “Indiana Jones,” the system might suggest “Jurassic Park” to you because it was well-received by users with similar tastes.
Content-Based Filtering
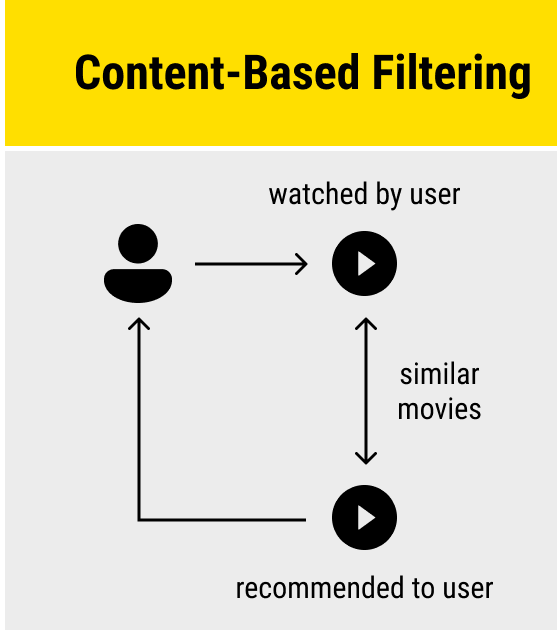
Content-based filtering recommends items to users based on the characteristics of the items and the user’s past interactions. For instance, in a music streaming service, if a user has liked rock songs in the past, the system might suggest more rock songs or artists with similar musical characteristics.
Let’s say you’re using a news app. Content-based filtering would recommend articles based on your past reading habits. If you often read technology articles, the system might suggest a new tech article that matches your interests, even if it’s from a different source.
Hybrid Recommender Systems
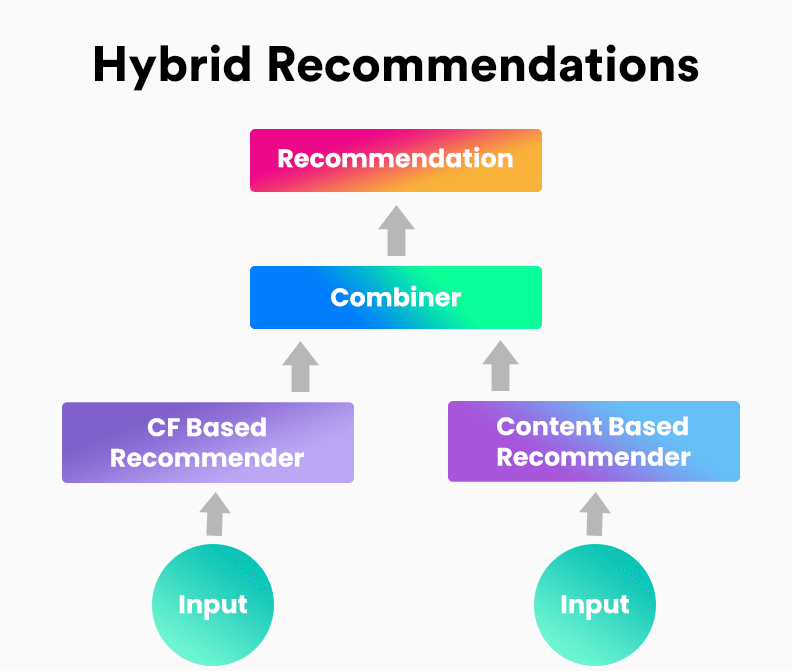
These systems combine multiple recommendation techniques to provide more accurate and diverse suggestions. For instance, a hybrid recommender system might use both collaborative filtering and content-based filtering to offer a wider range of recommendations.
Consider an e-commerce website like Amazon. Hybrid systems combine collaborative filtering and content-based filtering.
They might suggest products based on your past purchases (collaborative) and also consider the features of the products themselves (content-based) to offer a diverse set of recommendations.
Matrix Factorization
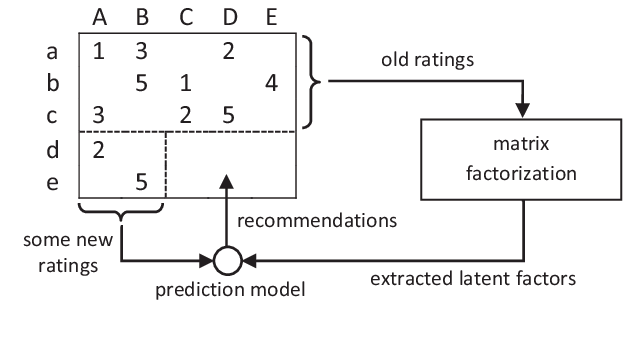
This approach decomposes user-item interaction data into latent factors and then makes recommendations based on these factors. It’s commonly used in recommendation systems for movies, where it can identify hidden patterns in user preferences.
In the context of music streaming, matrix factorization could break down your music preferences into latent factors. For example, it might discover that you like upbeat songs with catchy melodies, even if you’ve never explicitly stated that. It would then recommend songs with similar latent factors.
Contextual Recommender Systems
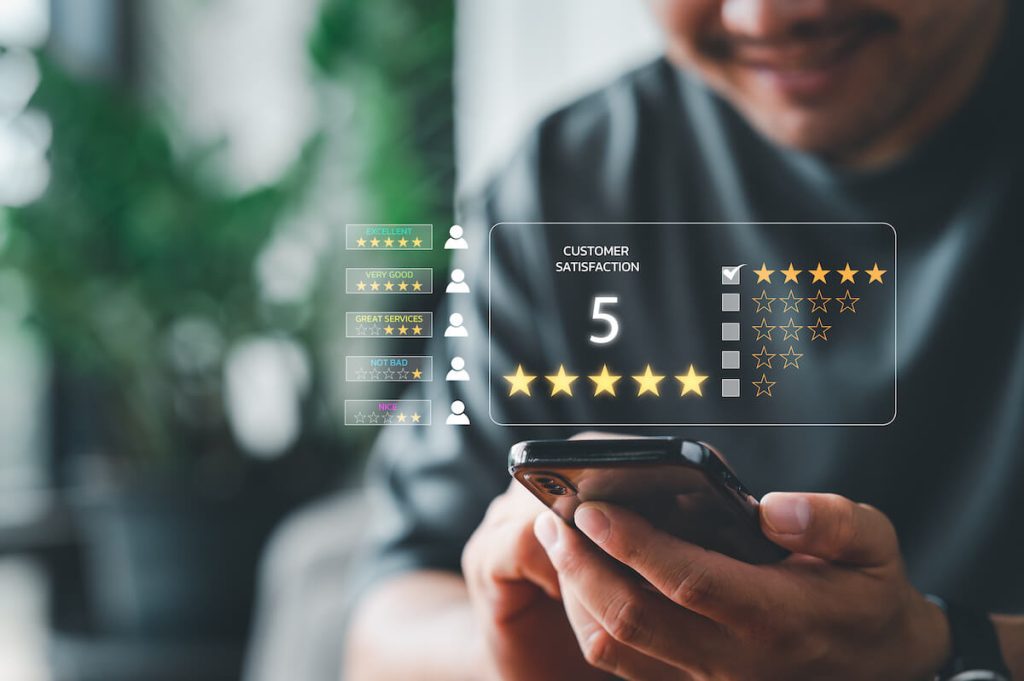
These systems consider the context in which recommendations are made, such as the user’s location, time, or device. For instance, a contextual recommender might suggest nearby restaurants based on a user’s current location.
Think about a weather app on your smartphone. A contextual recommender system would provide weather-related recommendations based on your current location. If it’s raining, it might suggest bringing an umbrella or looking up indoor activities.
Association Rule Mining
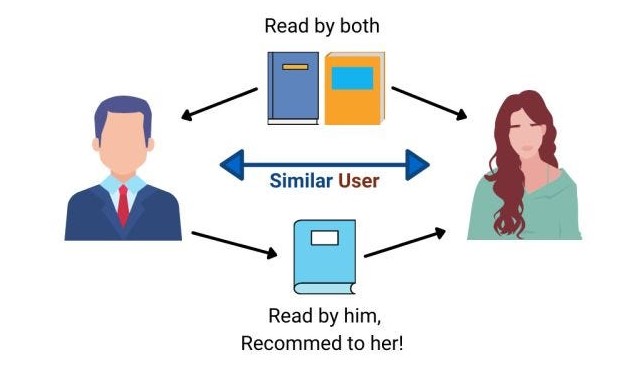
This technique identifies associations or patterns in user behavior. For example, it might discover that users who buy diapers are also likely to buy baby wipes, leading to recommendations for both products together.
In an online retail store, association rule mining might discover that customers who buy a laptop are likely to purchase a laptop bag as well. So, if you buy a laptop, the system could recommend laptop accessories like bags or mouse pads.
Deep Learning Recommender Systems
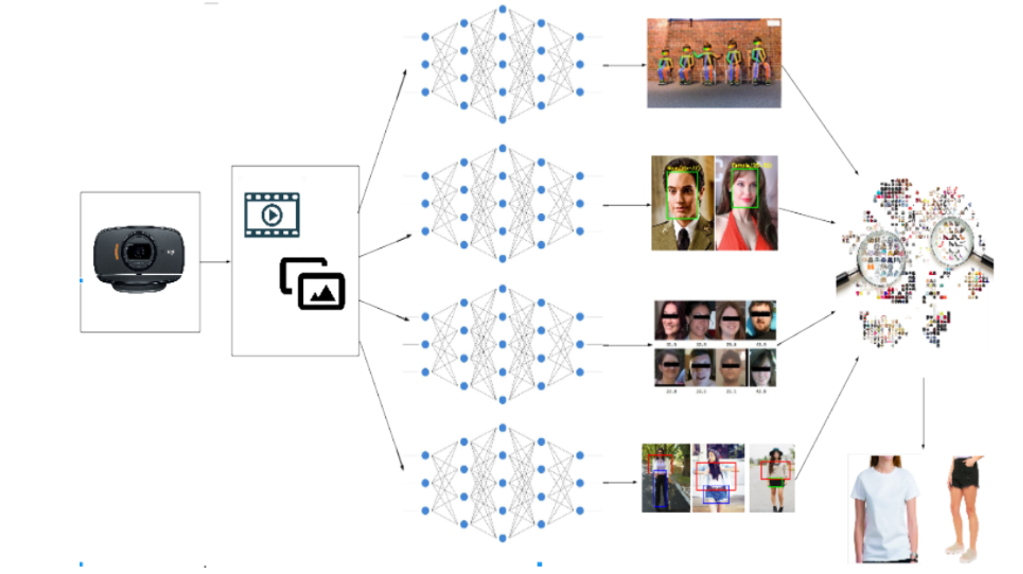
Deep learning models, such as neural networks, can be used to learn complex patterns in user data. These systems are capable of handling vast amounts of data and are often used by large-scale platforms like Netflix and Amazon.
Platforms like YouTube use deep learning models to analyze your video-watching history and recommend videos. Deep learning algorithms can identify intricate patterns in your viewing behavior and suggest relevant content.
Knowledge-Based Recommender Systems
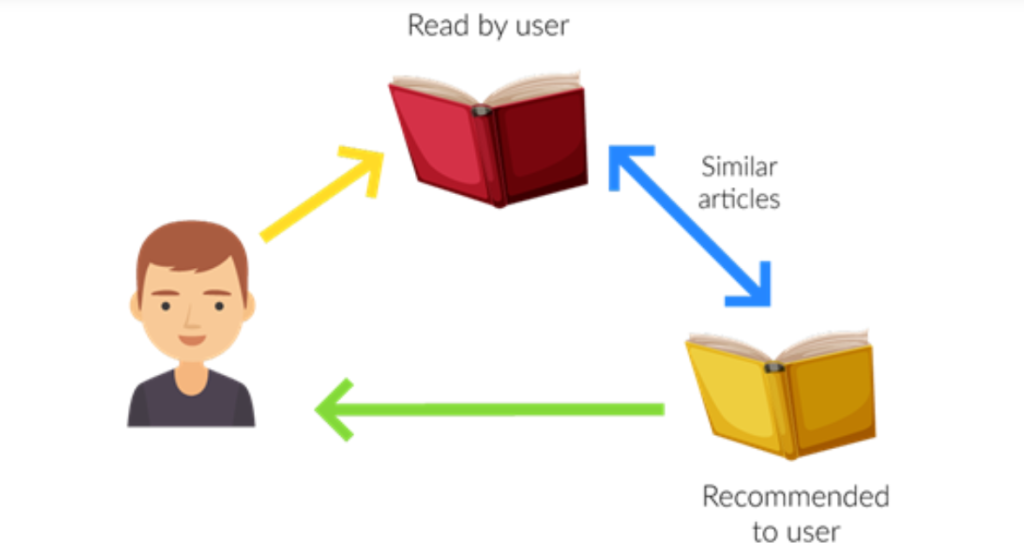
These systems rely on explicit knowledge about items and users. For instance, a real estate recommendation system might consider user preferences and property characteristics to suggest suitable listings.
Imagine you’re planning a vacation, and you provide information about your preferred travel dates, destination, and budget to a travel website. A knowledge-based recommender system would use this information to suggest hotels, flights, and activities that match your criteria.
Demographic-Based Recommender Systems
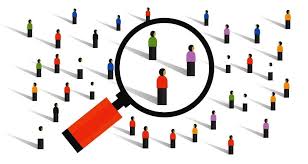
Recommendations are made based on demographic information such as age, gender, or location. For example, an e-commerce platform might suggest clothing items tailored to a user’s age group and gender.
In a music streaming app, a demographic-based recommender might recommend different playlists for users of different age groups. For example, it might suggest “Top Hits 2020” for younger users and “Classics from the ’80s” for older users based on their age demographic.
Implicit vs. Explicit Feedback Recommenders
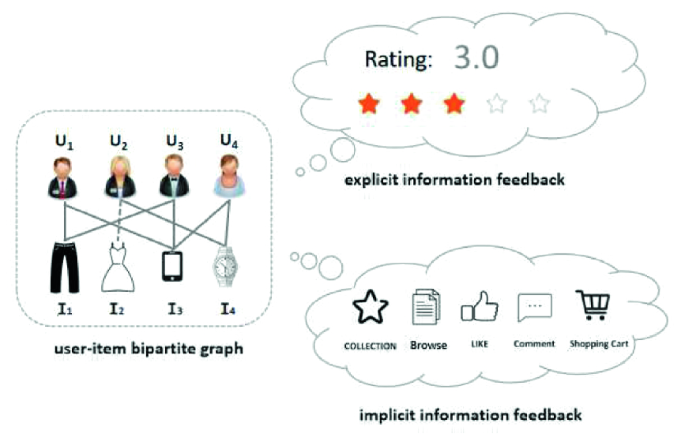
Recommender systems can use both implicit feedback (user behavior like clicks and views) and explicit feedback (user ratings and reviews) to make recommendations. This allows for more flexibility in the recommendation process.
Consider an e-commerce platform. Implicit feedback might involve analyzing a user’s browsing history and clicks to recommend products. Explicit feedback might involve asking users to rate products and then recommending items based on their ratings and reviews.
These are some of the main types of recommender systems, and they can be further customized and combined to suit the specific needs of different applications, whether it’s suggesting movies, products, music, or content.
Hybrid Recommender System
Delving into the realm of recommender systems, this section actively elucidates the concept of hybrid recommender systems, where multiple recommendation approaches are dynamically amalgamated. It goes beyond a passive description, actively emphasizing the advantages inherent in hybrid models and underscoring their pivotal role in mitigating limitations while actively enhancing the accuracy of recommendations.
Active Amalgamation of Approaches
In the exploration of hybrid recommender systems, there is an intentional and dynamic integration of diverse recommendation approaches. It involves the strategic blending of methodologies, ensuring an active synergy that combines the strengths of different models.
Emphasis on Advantages
This section doesn’t merely outline the concept; it actively emphasizes the advantages embedded in hybrid models. It goes beyond a passive acknowledgment, actively articulating how the combination of various recommendation approaches results in a more robust and effective system.
Mitigation of Limitations
The active engagement extends to addressing limitations inherent in individual recommendation approaches. Hybrid models are positioned as active solutions, strategically designed to overcome the shortcomings of singular methods, contributing to a more resilient and adaptable recommender system.
Improvement in Accuracy
The active role of hybrid models is underscored by their direct contribution to improving the accuracy of recommendations. This isn’t a theoretical assertion but an active demonstration of how the amalgamation of approaches leads to more precise and contextually relevant suggestions.
In essence, this section serves as an active guide to understanding and appreciating hybrid recommender systems, actively promoting their adoption as a strategic means to elevate the effectiveness of recommendation engines. The active voice ensures that the reader is not a passive observer but an engaged participant in the exploration of the dynamic landscape of hybrid recommender systems.
Data Collection and Preprocessing
Data Collection
We actively engage in acquiring user interactions and preferences as part of the data collection process for recommender systems, which is the cornerstone of how the systems function as a whole. This proactive participation frequently involves the use of user-item interactions, which might include things like product purchases, clicks, or ratings. Furthermore, we draw on both explicit feedback—such as user reviews—and implicit feedback—inferred from user behavior—both of which offer insightful information on user preferences.
The system’s capacity to accurately determine user preferences is improved by the active collection of various data kinds. We create a strong dataset that serves as the foundation for creating precise and efficient recommender systems by combining numerous aspects of user interactions and feedback, spanning from explicit to implicit sources. This broad approach to data collection not only ensures a comprehensive understanding of user preferences but also enriches the system’s ability to make nuanced and contextually relevant recommendations.
Data Preparation
After the data has accumulated, a proactive and planned strategy is adopted to methodically prepare the dataset, assuring its maximum compatibility with the advanced algorithms used in recommender systems. This crucial stage necessitates meticulous cleaning and pre-processing efforts to address the myriad problems that real-world datasets have by their very nature. A crucial element in boosting the dataset’s trustworthiness is the attentive attention paid to problems like missing values, outliers, and discrepancies within the dataset.
The need for raw data to be transformed into an organized and consistent format makes this process more than just a procedural step. This crucial procedure lays the foundation for later in-depth analysis and modeling, creating the opportunity to glean valuable insights. The willingness to actively participate in data preparation demonstrates a dedication to streamlining and standardizing the dataset to maintain its coherence and integrity.
This meticulous data preparation is more than just performing normal duties; it is an active, dynamic involvement. To improve the quality of the dataset, one must comprehend its complexities, use domain-specific knowledge, and make wise decisions. Structure and uniformity must be imposed for algorithms to run smoothly and for a thorough grasp of the underlying patterns in the data to be possible.
Active participation in data preparation goes beyond a simple technicality; it is an investment from a strategic standpoint. It prepares the ground for the efficient application of recommender system algorithms, making sure that the recommendations that follow are correct and indicative of the underlying dynamics in the data. Recommender systems use the refined and standardized dataset as a canvas to paint sophisticated and contextually appropriate recommendations.
Importance of Data Quality
It is impossible to overestimate the importance of data quality in the context of recommender systems. Accurate and trustworthy suggestions depend on the availability of high-quality data. When biases or inaccuracies in the data are propagated through the recommendation process, the results can be distorted and less than ideal. For the system to deliver accurate and useful recommendations, it is critical to ensure that the data is indicative of user preferences and behaviors.
Impact on Recommendations
The lifespan of a recommendation is affected by data quality. Incomplete or inaccurate data might cause the system to become noisy, making it more difficult to identify true user preferences. In consequence, this jeopardizes the precision and applicability of suggestions. A strong recommender system depends on a base of high-quality data to identify trends, correlations, and subtleties in user interactions, improving the accuracy of its recommendations.
Addressing Sparsity
When it comes to sparsity, a problem that frequently arises in recommender systems because consumers may only interact with a small portion of the available items, data quality is very important. High-quality data makes it easier to handle sparse datasets effectively, allowing the system to generate defensible deductions and recommendations even when there are few clear user-item interactions.
User Trust and Engagement
Beyond algorithmic factors, data quality is crucial for promoting user engagement and confidence. The overall user experience is improved when recommendations are based on precise, representative data since they are more likely to match the tastes of the users. On the other hand, unreliable recommendations brought on by bad data quality might damage user confidence and reduce the effectiveness of the system.
In essence, the development and functioning of recommender systems depend on the active collection and precise compilation of high-quality data. These procedures affect the entire recommendation pipeline, affecting the system’s accuracy, relevance, and, ultimately, its capacity to offer consumers useful and reliable recommendations.
Machine Learning Models
The complex world of machine learning models is at the core of recommender systems. The main area of concentration is this crucial domain, where various models essential to recommendation engines are actively explored. This section’s journey includes a thorough analysis of well-known models including matrix factorization, neural networks, and contemporary deep learning techniques.
The journey starts with a thorough investigation of matrix factorization, a process that analyses the user-item interaction matrix to extract latent characteristics and offer a sophisticated comprehension of underlying patterns. Neural networks, a mainstay of machine learning, are introduced as effective tools for understanding subtle relationships in data, demonstrating their effectiveness in identifying nuanced consumer preferences. The investigation goes further into modern deep learning techniques, which use complex structures to derive hierarchical representations from data, improving the ability to recognize complex connections and patterns.
A thorough comparative analysis that highlights the advantages and disadvantages of each model highlights the need for this investigation even more. This active evaluation is not just a list of features; rather, it is a calculated investigation that reveals the subtleties influencing each model’s effectiveness in the context of recommender systems.
For instance, matrix factorization excels at handling sparse data and extracting latent factors but may have scaling issues. Neural networks provide a reliable solution but may require a lot of computer power due to their ability for nonlinear mapping. While modern deep-learning techniques are effective at capturing complex patterns, they may need large amounts of data to operate at their best. This active research of machine learning models is more than just a theoretical investigation; it is an effort to comprehend the real-world applications and trade-offs related to each model. It explores the complicated dynamics that control how algorithms are applied in the vast field of recommender systems, going beyond a simple list of methods.
This section essentially acts as a dynamic guide, guiding the reader through the varied world of machine learning models and how each one adds to the complex tapestry of recommender systems. Because of the thoroughness of the investigation, the implementation of these models going forward will be guided by a thorough grasp of their capabilities and limitations. It places the investigation in a strategic framework, where choosing a model requires careful consideration in light of the unique needs and difficulties presented by the recommender system landscape.
Evaluation Metrics
In the realm of recommender systems, an active exploration unfolds, delving into the intricacies of evaluation metrics designed to assess system performance. This section meticulously dissects the specialized metrics crafted for recommendation algorithms, actively addressing the challenges and nuances inherent in evaluating the effectiveness of these systems.
The journey into evaluation metrics is not a passive overview but a dynamic engagement that seeks to unravel the complexities of measuring recommender system performance. It involves a strategic examination of metrics tailored specifically for recommendation algorithms, encompassing a diverse set of parameters that capture the nuances of user-item interactions.
The active scrutiny extends beyond a surface-level examination, venturing into the challenges associated with evaluating recommender systems. These challenges range from addressing the inherent biases within algorithms to navigating the intricacies of dealing with sparse data and diverse user preferences. The section doesn’t merely enumerate metrics but actively navigates through the landscape of potential obstacles, providing insights into mitigating strategies and nuanced approaches for effective evaluation.
By addressing challenges head-on, this exploration contributes to a more profound understanding of the evaluative process, recognizing that recommender systems operate in dynamic and evolving environments. The active voice in this exploration emphasizes a hands-on, participatory approach to dissecting metrics, fostering a comprehensive understanding of their applicability and limitations.
This section serves as an active guide, steering through the intricate terrain of evaluation metrics in recommender systems. It goes beyond a passive exposition, actively engaging with the metrics, challenges, and nuances, ensuring that the evaluation process is not just a routine task but a strategic endeavor to gauge the true effectiveness of recommendation algorithms.
Challenges in Recommender Systems
In navigating the landscape of recommender systems, challenges take center stage, with a focus on critical issues such as the cold start problem, sparsity, and scalability. This section actively places these obstacles at the forefront, conducting a thorough examination to uncover the intricate hurdles that recommender systems encounter in their deployment.
Cold Start Problem
The cold start problem arises when a recommender system faces difficulty providing accurate recommendations for new or unseen users or items. Imagine a new user signing up for a streaming platform with no historical interaction data. Conventional recommendation approaches may struggle to understand and cater to their preferences, posing a significant challenge.
Solution: Implementing content-based recommendations for cold-start users, relying on item characteristics or user profile information, can alleviate the cold-start problem by offering relevant suggestions based on available attributes.
Sparsity
Sparsity becomes a prominent challenge when the interaction matrix between users and items is predominantly empty, with users interacting only with a small fraction of available items. Consider an e-commerce platform where users tend to purchase or view only a limited set of products, resulting in a sparse matrix.
Solution: Techniques such as matrix factorization or collaborative filtering, which can handle sparse data by filling in missing values through latent factor representations, address the sparsity challenge effectively.
Scalability
Scalability issues emerge when recommender systems struggle to handle a growing number of users, items, or interactions. For instance, a popular e-commerce platform witnessing a surge in user activity may find it challenging to maintain real-time recommendation updates.
Solution: Employing distributed computing frameworks or scalable algorithms ensures that recommender systems can handle increased loads efficiently, providing timely and accurate recommendations even as the user base expands.
Ethical Considerations and Biases
Ethical considerations, particularly biases within algorithms, are scrutinized with an emphasis on fostering a comprehensive understanding of the challenges inherent in the deployment of recommendation engines. Biases may emerge when recommendations favor certain user demographics over others, leading to potential discrimination.
Solution: Actively implementing fairness-aware algorithms and incorporating diverse training data can mitigate biases, ensuring that recommendations are equitable and avoid reinforcing existing societal prejudices.
By actively addressing these challenges and proposing solutions, this section contributes to a nuanced understanding of the intricacies surrounding the deployment of recommender systems. It goes beyond mere identification, actively engaging with potential remedies to ensure that recommender systems are resilient in the face of real-world complexities.
Personalization Techniques
In the exploration of personalization techniques within recommender systems, this section actively delves into the intricacies of how these systems dynamically tailor recommendations to individual users. It goes beyond a mere surface-level explanation, providing a detailed understanding of the active processes involved.
Understanding User Preferences
At the core of personalization lies a meticulous understanding of user preferences. This section actively explores how recommender systems engage in the continuous analysis of user interactions and behaviors. It’s not a passive observation but an active process that involves discerning patterns, identifying preferences, and adapting in real time to the evolving tastes of individual users.
Dynamic Nature of Personalization Techniques
The term “dynamic” here is not incidental. This section actively emphasizes the ever-changing nature of personalization techniques. Recommender systems don’t adopt a static approach; rather, they actively evolve based on user feedback and behavior. This involves real-time adjustments to recommendations, ensuring that users receive content that aligns with their current preferences, making the personalization process a continuous and adaptive journey.
Tailoring Recommendations
The active voice in this section is crucial in conveying the proactive nature of recommender systems. It’s not a passive delivery of recommendations but an ongoing, participatory process. Recommender systems actively tailor recommendations by leveraging user history, preferences, and even contextual information. For example, if a user suddenly shows a strong interest in a new genre, the recommender system actively incorporates this information to adjust future recommendations.
User-Centric Focus
This section actively underscores the paramount importance of a user-centric approach. It’s not just about generating recommendations; it’s about enhancing the user experience. Recommender systems actively prioritize user satisfaction by tailoring recommendations to align with individual preferences, creating a personalized journey that resonates with each user.
Advanced Techniques
The exploration of personalization techniques extends beyond basic approaches. This section actively delves into advanced methodologies, such as collaborative filtering, content-based filtering, and hybrid models. It’s not a superficial overview but an in-depth examination of the algorithms and strategies that actively contribute to the personalized recommendations users experience.
In essence, this section is an active guide, navigating through the complexities of personalization in recommender systems. It’s not a passive observation but an engaging exploration that aims to convey the dynamic, proactive, and user-centric nature of personalization techniques. By actively explicating these intricacies, it provides a nuanced understanding of how recommender systems actively shape and enhance user experiences through personalized recommendations.
Real Time Application of Recommender System
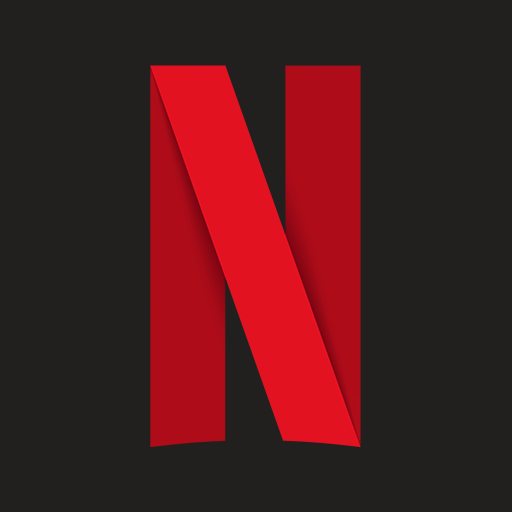
- Netflix Recommendation System
- Netflix, a leading streaming platform, employs a sophisticated recommender system to enhance user experience. This system actively analyzes user viewing habits, preferences, and interactions with content. It goes beyond a mere list of movies or shows, actively tailoring recommendations based on intricate patterns in user behavior. For instance, if a user frequently watches science fiction movies, the recommender system dynamically suggests similar genres, providing a personalized and engaging streaming experience. The active analysis of user data ensures that recommendations align with individual tastes, making the platform a powerhouse in the streaming industry.
- Amazon Product Recommendations
- Amazon, a global e-commerce giant, utilizes a robust recommender system to personalize product suggestions for users. This system actively scrutinizes users’ purchase history, browsing behavior, and even demographic information to offer tailored recommendations. For instance, if a user has been exploring fitness equipment, the recommender system dynamically suggests related products such as workout gear, supplements, or fitness trackers. This active personalization not only enhances user satisfaction but also contributes to increased sales by presenting users with items aligned with their interests and needs. Amazon’s recommender system serves as a prime example of how active data analysis can significantly impact user engagement and business outcomes.
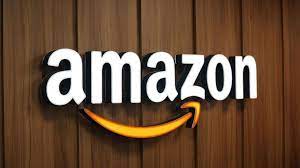
Conclusion
recommender systems play an indispensable role in the modern digital landscape, actively shaping user experiences, boosting engagement, and driving business success through personalized recommendations. As these systems continue to evolve, their ability to harness data, apply advanced models, and address ethical considerations will be pivotal in delivering accurate, user-centric suggestions that cater to individual preferences, ultimately enhancing the way we interact with digital platforms and services.