Neural Style Transfer: Power of AI in world of ART
What is art?
We have our own definitions of art in many ways. For many people, art is an imaginary boundary that manifests its images in multiple ways.
For some, art is a human expression that creates emotions, tells stories, and explores the imagination of everything.
As per me, “Art is a wonderful and powerful part of human civilization that involves the use of various elements and principles such as colour, line, shape, texture, and rhyme that completely balance an artist.
What is Art in AI?
Let me define “art in AI” as per the image attached above.
Art in AI is the combination of artificial intelligence (AI) and artistic creativity. It involves the application of AI techniques, such as machine learning, deep learning, and neural networks, in the creation, analysis, and interpretation of various forms of art.
Art has always been a powerful form of human expression that conveys emotions, tells stories, and explores our imagination. As AI grows along with the advancements in generative artificial intelligence (AI), it has been proven that if we merge AI and art together, we can create a wonderful application called Neural Style Transfer, a technique that enables the transfer of artistic style from one image to another. In this blog, we will delve into the world of neural style transfer, exploring how generative models can create unique and visually appealing artwork.
What is Neutral Style Transfer
I will define and explain the neutral style transfer with an example:
Let’s take an example if we have two images, lets combine the content of one image with the other image that result in an entirely new composition. It involves the extraction of features from the content image and the style image.
The objective is to minimize the difference between the content features of the generated image and the content features of the content image, while simultaneously minimizing the difference between the style features of the generated image and the style features of the style image.
Backbone of generative AI Model:
The backbone of a generative AI model typically involves the underlying architecture or network structure that forms the foundation of the model. It includes the generating new content, like images, text, music, or other forms of data, based on the patterns and knowledge.
The choice of backbone architecture depends on the specific task and requirements of the generative AI model.
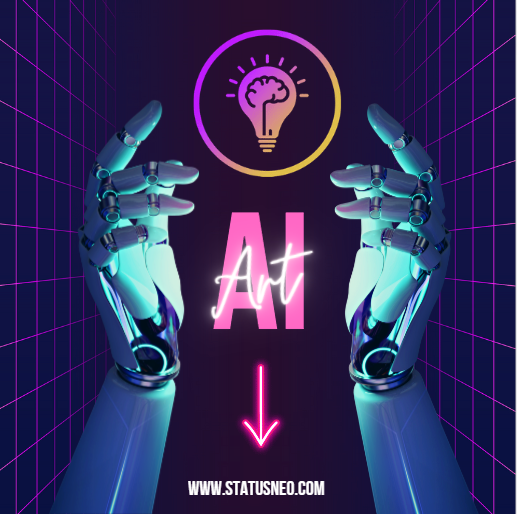
Generative Adversarial Networks (GANs):
GANs consist of two components, a generator, and a discriminator, which are trained together in a competitive setting.
Now, what does a generator does?
The generator tries to produce content that is different from real data, while the discriminator’s role is to differentiate between real and generated data.
We can apply GANs to tasks such as image generation, style transfer, and text generation.
In the above image, it is clear how beautifully Generative Adversarial Networks are actually generating images through Generator and then discriminating the real faces.
Variational Autoencoders (VAEs):
It consists of an encoder and decoder. The encoder maps input data into a latent space, where the decoder reconstructs the data. It aims to learn the distribution of input data and generate new samples from that distribution.
Transformers:
Transformers play significant role in natural language processing (NLP) tasks, including text generation and language translation. It includes the utilization of self-attention mechanisms to capture dependencies between different words or tokens in the input sequence.
Recurrent Neural Networks (RNNs):
RNNs are widely used in sequential data generation tasks, such as text generation and music composition. They process input data sequentially, maintaining internal memory to capture contextual information. Variants of RNNs, such as Long Short-Term Memory (LSTM) and Gated Recurrent Units (GRU), have been employed to generate realistic and coherent sequences.
Magic Behind the Neutral Style Transfer:
The magic behind the neutral style transfer is utilizing a pre-trained CNN, such as VGGNet or ResNet, as the backbone of the generative model. These networks are trained on vast datasets. By selecting specific layers of the network, we can capture different levels of abstraction. The lower layers capture the low-level details, such as edges and textures, while the higher layers capture more complex structures and semantic information.
The process involves the following steps:
- Generated Image initialization: The starting point would be the content image or the noise image. We can generate the image through the backbone of the Gnerative AI.
- Defining the loss functions: This feature actually measures the difference between the content features of the generated image and the content image. Style loss measures the difference between the style features of the generated image and the style image.
- Optimization of the generated image: The generated image is updated iteratively using optimization techniques such as gradient descent, minimizing the combined content and style losses.
- Fine-tuning the parameters: The hyperparameters, such as the weights for content and style losses, can be adjusted to achieve the desired artistic effect.
The Impact and Future:
The combination of AI and art has made the revolutionizing creative expression. It not only allows artists to explore new artistic styles and techniques but also provides an avenue for individuals without formal artistic training to engage in the creative process. Moreover, this technique can be utilized in various domains, including graphic design, advertising, and entertainment industries, to create visually captivating content that grabs attention and evokes emotions.
Final Intake:
Neural Style Transfer is an application of generative AI that brings together the worlds of art and technology. We can enable the transfer of artistic style from one image to another, and with the help of this functionality of Generative AI, we can create as many as applications and also generated many real images.
I just tried to explain the function or application of generative AI. I hope this will help us have something new and innovative.
Add Comment
You must be logged in to post a comment.