Leveraging AI and Machine Learning in Cyber Security
Strengthening Defenses in the Digital Age
In the continuously changing world of cyber threats, enterprises are increasingly relying on artificial intelligence (AI) and machine learning (ML) technologies to strengthen their cyber security defenses. These new techniques provide a proactive approach to threat identification and response, allowing security teams to remain ahead of attackers. This blog investigates the role of AI and ML in cyber security, analyzing their benefits, goals, problems, and transformative impact on digital asset protection.
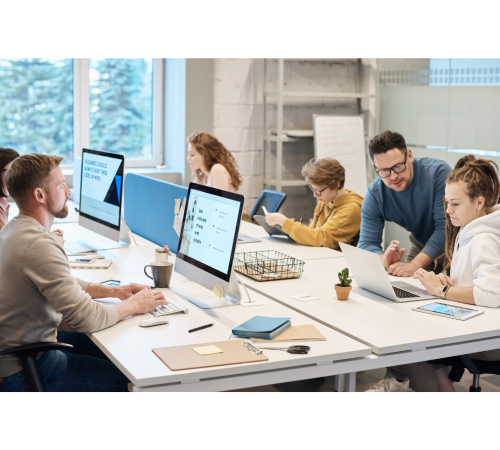
But the main question is WHY AI? Weren’t the earlier methods good?
Before the widespread use of AI products and services in enterprises, the daily job atmosphere was defined by manual processes, restricted automation, and traditional methods of functioning. Here is a brief outline of the scenario before the introduction of AI into industrial workflows:
- Manual Processes: Industrial operations relied primarily on manual labor for duties including assembly line operations, quality control inspections, and inventory management. This frequently resulted in inefficiencies, errors, and increased labor expenses.
- Restricted Automation: While some automation existed in certain industrial processes, it was frequently restricted to simple tasks and lacked the depth and intelligence provided by AI-powered systems. Automation was largely concerned with repetitive and mechanical chores, rather than complicated decision-making processes.
- Predictive Maintenance Challenges: Maintenance activities were mainly reactive, with equipment faults and failures resulting in costly downtime and output losses. Predictive maintenance solutions, which rely on data analytics and machine learning, have not been widely used, resulting in inefficiencies and greater maintenance costs.
- Quality Control Issues: Quality control inspections were based on manual inspections and sample methods, which were prone to human error and inconsistency. Detecting faults and anomalies in real time was difficult, resulting in quality control concerns and creating the product again, from scratch, thus wasting time and money.
- Data Management Challenges: Industrial data was frequently separated, divided, and kept in multiple systems, making it difficult to extract useful insights and make informed choices. Traditional analytics solutions could not manage enormous amounts of data while providing real-time insights.
All these lead to the usage of a far better, more efficient, resource-saving tool called Artificial Intelligence!
Impact After the Usage of AI Tools and Services
- Increased Efficiency: AI-powered automation improves industrial workflows, eliminating the need for manual labor while enhancing operational efficiency. Tasks that formerly required human intervention, such as predictive maintenance scheduling and inventory optimization, have now been automated, resulting in cost savings and increased productivity.
- Enhanced Predictive Maintenance: AI-enabled predictive maintenance systems evaluate equipment sensor data in real-time to detect potential breakdowns before they happen. This proactive strategy decreases downtime, increases equipment durability, and lowers maintenance costs by scheduling maintenance activities according to actual equipment conditions rather than fixed schedules.
- Improved Quality Control: AI-based quality control systems use machine learning algorithms to detect flaws and abnormalities in real-time, maintaining consistent product quality and lowering the risk of problems entering the market. Computer vision and image recognition technologies enable automated visual inspections, which improves the accuracy and efficiency of quality control operations.
- Data-driven decision-making: With AI tools and services, industrial organizations can leverage the power of big data and complex analytics to extract actionable insights from massive amounts of data. Real-time and predictive analytics skills enable decision-makers to make more informed decisions, optimize processes, and find new business prospects.
- Safety and Risk Management: AI technologies such as machine learning and natural language processing are used to assess safety data, detect possible dangers, and forecast safety risks in industrial settings. This proactive approach to safety management helps to prevent accidents, reduce workplace injuries, and assure compliance with safety standards.
Conclusion
Overall, the use of AI tools and services in industrial workflows has changed traditional industrial processes, resulting in increased productivity, better quality control, streamlined supply chain management, data-driven decision-making, and improved safety and risk management. By embracing AI, industrial organizations can remain competitive in the digital age and create innovation in their particular industries.