Unveiling the Power of Generative AI in Data Analytics and Business Intelligence for Epidemic Prevention and Outbreak Control
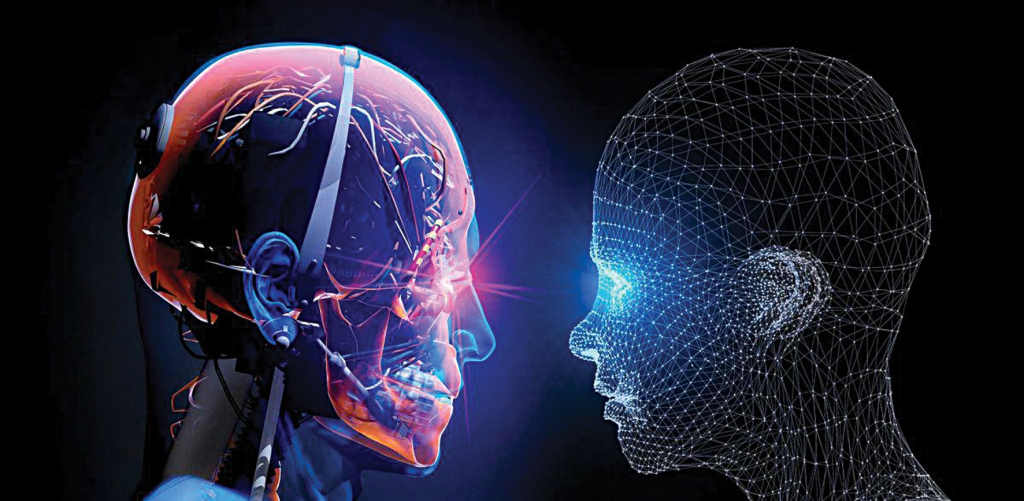
Introduction
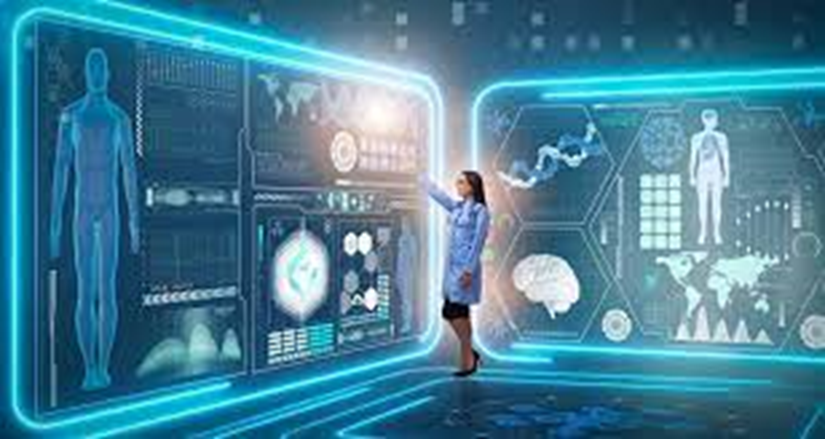
In the ever-evolving digital landscape, artificial intelligence (AI) has emerged as a formidable tool in various fields, including data analytics and business intelligence. With its remarkable capacity to analyze large volumes of data and extract valuable insights , AI holds immense potential in the domain of epidemic prevention and control.
As an epidemiologist, I am enthusiastic about exploring the transformative capabilities of generative AI in revolutininzing strategies for epidemic prevention, outbreak management, and planning. This blog aims to delve into the ways in which AI can effectively harness data, uncover meaningful insights, and pave the way for innovative approaches to address epidemics.
Unleashing the Power of Data
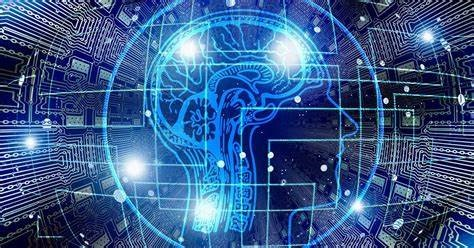
At the heart of epidemic surveillance and response lies the vital role of data. However, the exponential growth of data sources, such as electronic health records, social media platforms, and sensor networks, poses a significant challenge in extracting actionable information from these vast and intricate datasets. This is precisely where AI, particularly generative AI, emerges as a valuable ally, enabling us to unlock the untapped potential of data and drive proactive measures for epidemic prevention and outbreak management.
Harnessing Generative AI for insights
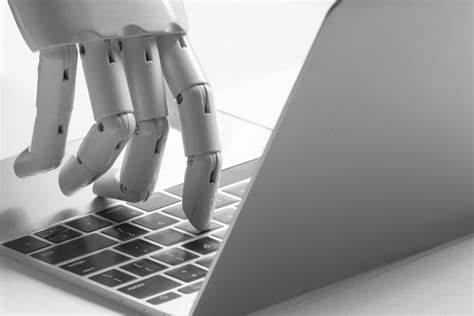
Generative AI algorithms have the capability to analyze and learn patterns from diverse datasets, generating new and meaningful insights. By applying generative AI techniques to epidemic data, we can unlock hidden patterns, identify potential outbreak hotspots, and even predict the spread of diseases in real-time. These insights provide crucial information for early detection, rapid response, and effective resource allocation.
Early detection and rapid response
One of the key benefits of AI in epidemic prevention is its ability to identify early warning signs. By analysing various data streams, AI algorithms can detect anomalies or unusual patterns that may indicate the emergence of a new disease or an outbreak. This enables public health officials to respond swiftly, implementing preventive measures, and containing the spread before it escalates.
Predictive Modelling and Risk Assessment
Generative AI techniques, such as machine learning and deep learning, can develop robust predictive models for epidemic outbreaks. By training on historical data, AI algorithms can identify risk factors, predict the spread of diseases, and estimate the potential impact on vulnerable populations. These models can aid policymakers in making informed decisions, allocating resources, and implementing targeted interventions to prevent the escalation of an epidemic.
Real time Surveillance and Monitoring
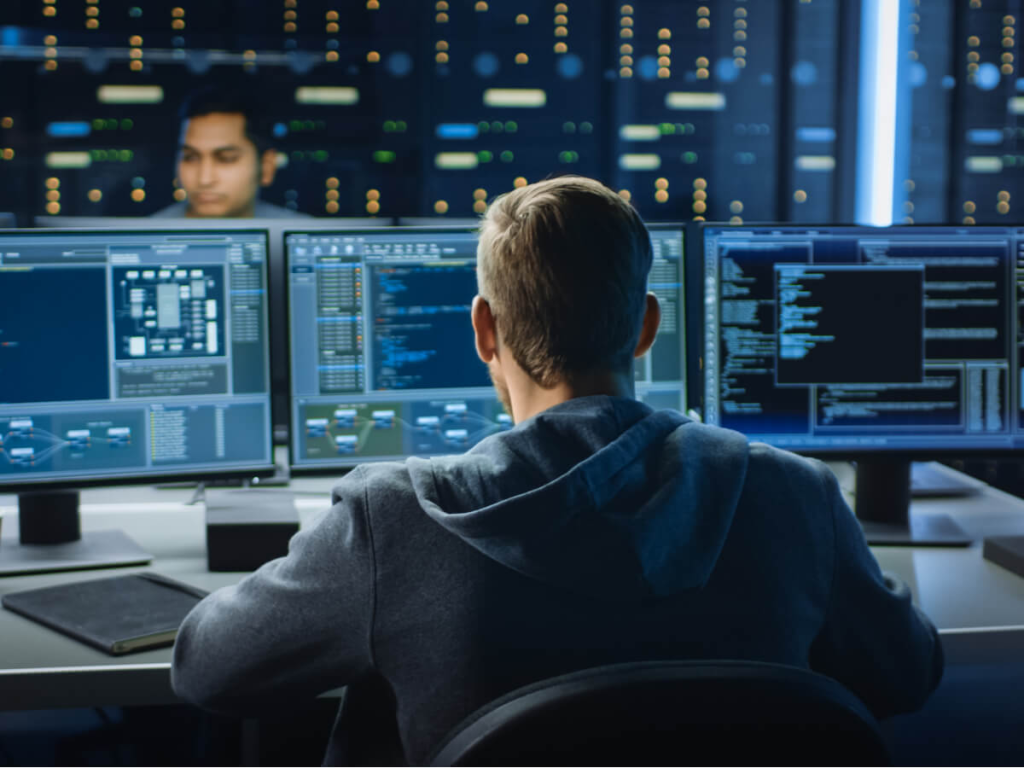
Generative AI systems can continuously monitor various data sources, including social media feeds, hospital records, and environmental sensors, providing real-time updates on disease prevalence, symptoms, and geographical patterns. By integrating this information into public health surveillance systems, authorities can gain a comprehensive understanding of the current situation, enabling targeted interventions and resource allocation.
Optimizing resource allocation
During epidemics, resource allocation becomes critical. AI algorithms can analyze historical data, assess risk factors, and predict the demand for healthcare services, medical supplies, and personnel. This information helps policymakers and healthcare providers optimize resource allocation, ensuring efficient and effective response strategies.
Simulating and planning outbreaks
Generative AI can simulate and model outbreaks, considering various parameters such as population density, mobility patterns, and disease characteristics. These simulations enable public health officials to evaluate different intervention strategies, assess their potential impact, and make informed decisions on the most effective measures to control and contain the outbreak.
Challenges with application of generative AI in epidemiological practices
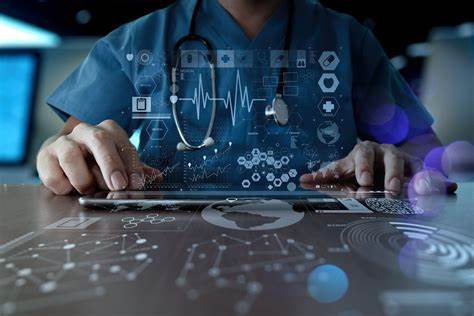
Integrating generative AI into preventive healthcare holds immense potential by leveraging deep learning models to generate synthetic data. However, the effective incorporation of this technology into epidemiological practises requires addressing various challenges and limitations.
- Unstructured Nature of Medical Data: One primary obstacle stems from the unstructured nature of medical data, which often lacks standardized annotations. The quality of AI algorithm models heavily relies on high-quality data, and compromised data quality poses a risk of inaccurate predictions and diagnosis, potentially impacting public health.
- Accurate labelling of Synthetic Data: The precise labelling of synthetic data is crucial as machine learning models ‘reliability depends on the quality of the data they are trained on. Synthetic data currently falls outside the scope of standard data protection laws, raising concerns about unauthorized dissemination beyond organizational boundaries. Misuse of synthetic data, presented as real data in clinical contexts, could have severe implications for patient well-being.
- Transparency and Interpretability of Algorithms: Ensuring transparency and interpretability of algorithms is another challenge affecting trust in AI with medical settings. Inadequate transparency hinders patient and physician acceptance of the technology, making it difficult to embrace its use in clinical practise. Addressing algorithmic errors and security vulnerabilities is crucial to mitigate risks and build trust.
- Cost of adoption: The healthcare industry faces significant challenges in terms of the cost associated with adopting generative AI software. Expenses and the scarcity of skilled professionals proficient in operating this technology impede market growth and prevent healthcare providers from fully capitalizing on the benefits of generative AI. Additionally, training generative models is costly, requiring access to GPUs, and synthetic data must balance realism and differentiation to prevent the identification of original data points. Establishing recognized metrics to measure the fidelity and anonymity of synthetic data becomes imperative to ensure realistic and anonymous data generation while safeguarding patient privacy. These metrics will further facilitate the training of machine learning models, advancing the field of healthcare.
Overcoming these challenges and limitations is essential for the successful integration of generative AI in healthcare. Doing so will pave the way for improved patient care and innovative applications in medical practice.
Conclusion
Generative AI presents a remarkable opportunity for transforming the way we prevent, detect, and respond to epidemics and pandemics. By harnessing the power of data analytics and business intelligence, AI can provide invaluable insights that aid in early detection, real-time surveillance, optimized resource allocation, and strategic outbreak planning.
As an epidemiologist, I am excited to witness the continued advancements in AI and its pivotal role in safeguarding public health.
Remember, while AI is a powerful tool, it is not a panacea. It is crucial to ensure that the ethical considerations, privacy concerns, and transparency remain at the forefront of AI development and deployment. By harnessing AI’s potential responsibly, we can truly harness its power to save lives and build a healthier future.