The Impact of Agentic AI on Data Privacy
Have you ever thought about what happens when the machines we build start making their own decisions? It’s happening right now with something called Agentic AI. These systems are far from ordinary—they autonomously process and react to data, making independent choices that were once the domain of humans. This capability marks a significant shift, enhancing operational efficiencies and driving innovations at an unprecedented pace.
This growing autonomy raises crucial questions: How do we maintain control over AI that can make its own choices about data? What mechanisms need to be in place to ensure that these decisions do not compromise individual privacy?
Understanding Agentic AI
Unlike conventional AI, which follows strict, predefined rules, agentic AI systems can make decisions autonomously based on their data analysis. These systems employ advanced machine learning techniques, allowing them to learn from their environment and adapt without human intervention.
A key feature of agentic AI is its goal-oriented functionality. Programmed with specific objectives, these AIs can independently navigate complex scenarios to achieve set goals. This capability is evident in applications ranging from optimizing supply chains to personalizing user experiences and even in medical diagnostics. As agentic AI becomes more integrated into various sectors, it’s crucial to understand its operational mechanisms and potential impacts, particularly in relation to data privacy.
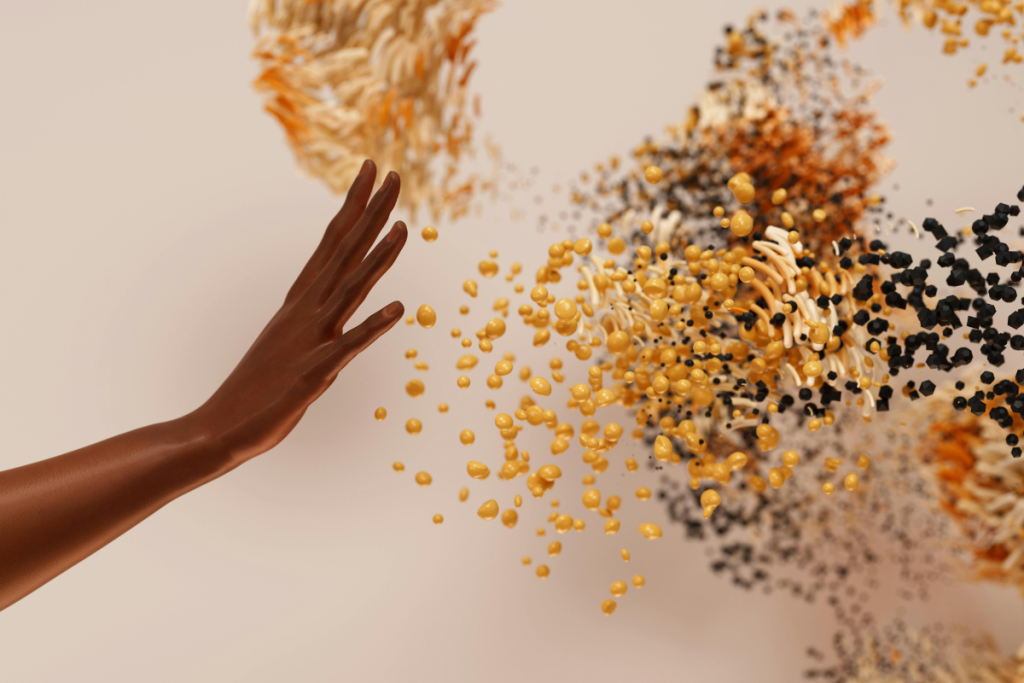
Here are some examples of agentic AI applications across various industries:
Healthcare
- Diagnostic Systems: Agentic AI is used in diagnostic applications that can analyze medical images, like X-rays or MRIs, to detect abnormalities such as tumors or fractures without human oversight.
- Personalized Treatment Plans: AI systems can review a patient’s history and ongoing health data to suggest customized treatment plans, adjusting them as new data becomes available.
Finance
- Fraud Detection: AI systems in finance autonomously monitor transaction patterns to identify and act on suspicious activities, reducing the risk of fraud.
- Investment Strategies: Agentic AI can manage portfolios by autonomously buying and selling stocks based on real-time market data and trends analysis.
Retail
- Personalized Marketing: AI systems analyze consumer behavior and preferences to tailor marketing messages and product recommendations autonomously.
- Supply Chain Optimization: AI optimizes inventory levels and logistics based on predictive analytics concerning demand forecasts and supply conditions.
Manufacturing
- Predictive Maintenance: AI systems monitor equipment conditions and automatically schedule maintenance tasks to prevent downtime, based on predictive analytics.
- Production Planning: AI can autonomously adjust production schedules and processes in response to supply chain fluctuations or demand changes.
Transportation
- Autonomous Vehicles: Self-driving cars and drones use AI to navigate and make real-time decisions about routes, speed, and safety.
- Traffic Management Systems: AI applications manage traffic flow based on real-time data, adjusting traffic signals and routes to optimize flow and reduce congestion.
Customer Service
- Chatbots and Virtual Assistants: These AI systems can handle a wide range of customer service queries without human intervention, learning from each interaction to improve their responses.
- Automated Problem Resolution: AI can identify customer issues from service tickets and autonomously provide or enact solutions.
Energy
- Grid Management: AI systems autonomously manage energy distribution based on real-time supply, demand, and price data, improving efficiency and stability of power grids.
- Renewable Energy Optimization: AI optimizes the operation of renewable energy sources, dynamically adjusting to weather conditions and energy demand.
Data Privacy Concerns with Agentic AI
As agentic AI systems become more prevalent across various sectors, they pose unique challenges to data privacy due to their autonomous decision-making capabilities. These systems often process large volumes of personal and sensitive data to learn and make informed decisions, which raises several privacy concerns:
- Autonomous Data Collection: Agentic AI systems can independently decide what data to collect, how long to retain it, and when to delete it. This autonomy can lead to the collection of more data than necessary, or the retention of data longer than required, without explicit consent from the individuals affected.
- Lack of Transparency: The decision-making processes of agentic AI are often based on complex algorithms that can be difficult for even the developers to understand, commonly referred to as the “black box” problem. This lack of transparency can make it challenging for users to know how their data is being used or to contest decisions made by AI.
- Potential for Bias: If not carefully managed, agentic AI can develop biases based on the data it is fed. These biases can lead to discriminatory practices, such as unfair denial of services or targeting in marketing, which can infringe on privacy and lead to broader social inequalities.
- Security Vulnerabilities: The autonomous nature of agentic AI makes it a prime target for cyber attacks. If compromised, agentic AI systems could misuse the data they control, or worse, make decisions that could endanger user privacy and safety.
- Compliance Challenges: With regulations like GDPR in Europe and CCPA in California, businesses are required to ensure transparent and accountable data processing practices. Agentic AI’s autonomous operations can complicate compliance efforts, as it may not always be clear who is responsible for the AI’s data handling practices.
- Informed Consent: Traditional concepts of informed consent are based on clear communication between data collectors and users. Agentic AI complicates this dynamic, as it may be challenging to provide users with clear, understandable information about what data the AI will collect, how it will be used, and what controls the user has.
So How Do We Proceed?
Navigating the complexities of agentic AI requires a strategic approach to balance innovation with responsibility. Below are essential solutions and best practices that can guide organizations in deploying these technologies effectively while ensuring data privacy and ethical integrity:
Robust Data Governance: Establish comprehensive data governance frameworks that outline clear policies on data usage, privacy, and security. This includes defining who has authority over data decisions and how data is collected, stored, and processed by AI systems.
Enhanced Transparency Measures: Increase the transparency of agentic AI systems through documentation and open communication about how the AI operates, the data it uses, and the decisions it makes. This could involve adopting standards for explainable AI that make it easier for users to understand and trust AI decision-making processes.
Ethical AI Protocols: Develop and enforce ethical guidelines specifically designed for agentic AI. This includes ensuring fairness, accountability, and absence of bias in AI algorithms. Regular audits and updates to these protocols can help address new ethical challenges as they arise.
Privacy by Design: Incorporate privacy considerations at every stage of the AI development process. This approach ensures that privacy is not an afterthought but a foundational component of agentic AI systems. Techniques such as data anonymization, encryption, and secure access controls should be standard practice.
Continuous Education and Training: Equip teams with the necessary skills and knowledge to manage and oversee agentic AI systems responsibly. This includes training in ethical AI practices, data privacy laws, and the technical skills needed to implement and maintain AI systems securely.
Stakeholder Engagement: Engage with all stakeholders, including users, regulators, and the public, to gain insights into their concerns and expectations. This engagement can inform the development of AI systems that are socially acceptable and beneficial.
Regulatory Compliance: Stay updated with current and emerging regulations related to AI and data privacy. Compliance should be monitored continuously, and AI systems should be adapted promptly to meet these regulatory requirements.
Incident Response Plans: Develop robust incident response plans that can quickly address any issues that arise with AI systems, such as data breaches or failures in decision-making. These plans should include procedures for containing incidents, assessing impacts, and communicating with affected parties.
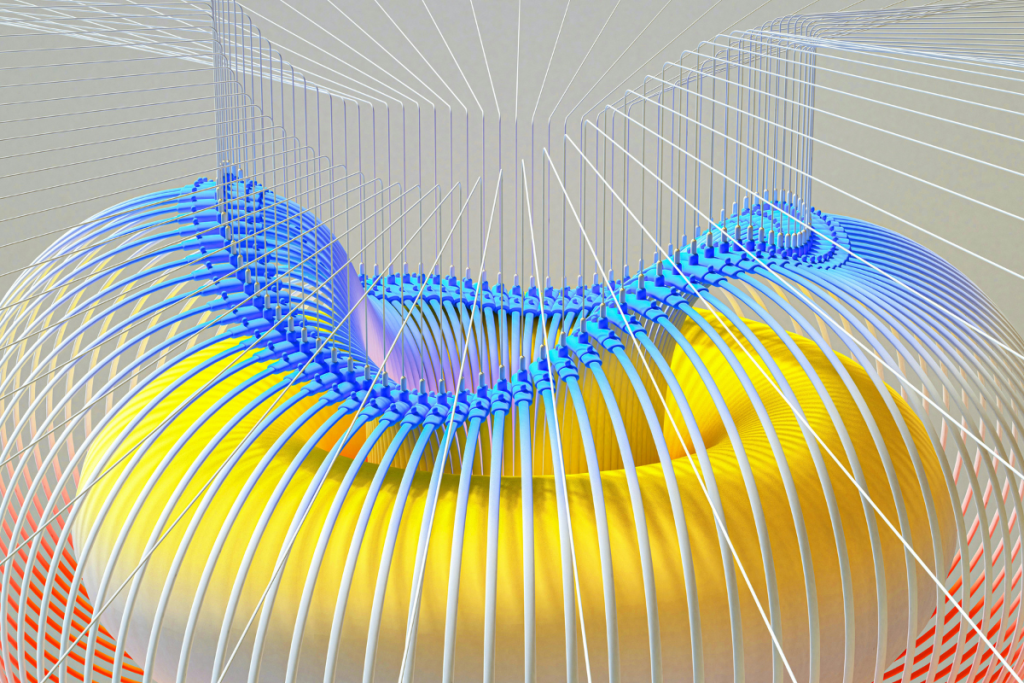
To Conclude
As we continue to integrate agentic AI into our daily lives, it’s vital to remember the bigger picture. This isn’t just about what AI can do; it’s about how we manage it responsibly. By adopting comprehensive best practices, enhancing transparency, and engaging with regulatory changes, we can safeguard data privacy and uphold ethical standards. This proactive approach ensures that agentic AI fulfills its promise of advancing our capabilities without compromising our values. Ultimately, the goal is to foster an environment where technology progresses in harmony with human interests, guided by a commitment to responsibility and respect for individual rights.
Check out my blog on A Beginner’s Guide to Behavior-Driven Development (BDD) and Business and Functional Focus in Behavior-Driven Development
For more such content make sure to follow us on LinkedIn