Secure and Smart: Leveraging Generative AI for Cybersecurity Solutions
One such cutting-edge technology that has emerged as a game-changer in the cybersecurity realm is generative artificial intelligence (AI). By harnessing the power of generative AI, cybersecurity professionals can enhance their defenses and respond more effectively to emerging threats.
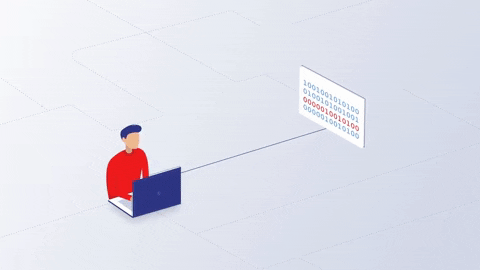
The evolving cybersecurity landscape:
In today’s interconnected world, where digital technologies permeate every aspect of our lives, cybersecurity has become a critical concern for individuals, businesses, and governments. The threat landscape is constantly evolving, with malicious actors employing sophisticated techniques to exploit vulnerabilities and compromise data security. Traditional cybersecurity measures often struggle to keep pace with these rapidly changing threats, making it imperative to explore innovative approaches that can enhance defense capabilities.
The role of generative AI in addressing emerging threats:
Generative artificial intelligence (AI) has emerged as a powerful tool in various domains, including cybersecurity. By harnessing the capabilities of generative AI, cybersecurity professionals can gain new insights, improve threat detection and response, simulate realistic attack scenarios, and train next-generation cybersecurity professionals. This blog post aims to delve into the applications and benefits of generative AI in the field of cybersecurity.
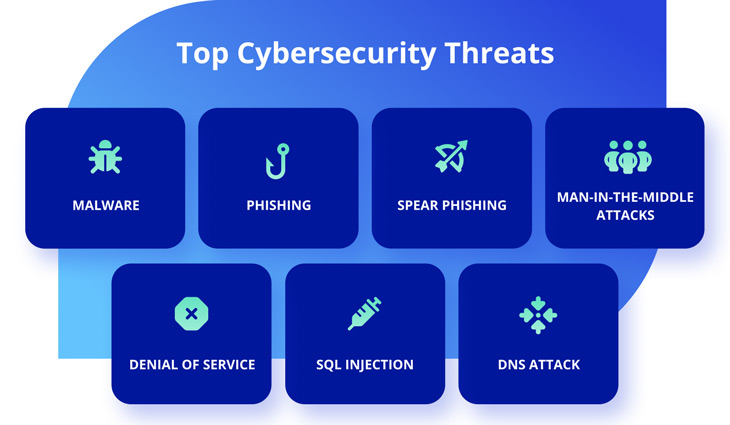
Understanding Generative AI For Cyber Security
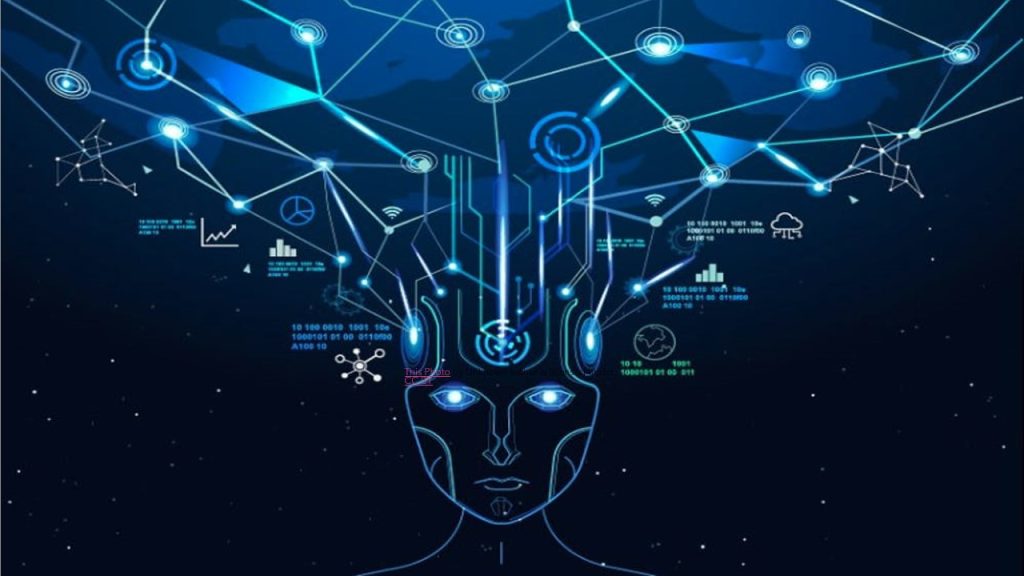
Overview of generative AI and its applications: Generative AI is a class of AI models that have the ability to generate new data samples that resemble the training data they were exposed to. This section provides an overview of generative AI, explaining its underlying principles, and highlighting its potential applications in cybersecurity. From generating synthetic data to creating realistic content, generative AI can offer valuable capabilities to augment existing cybersecurity solutions.
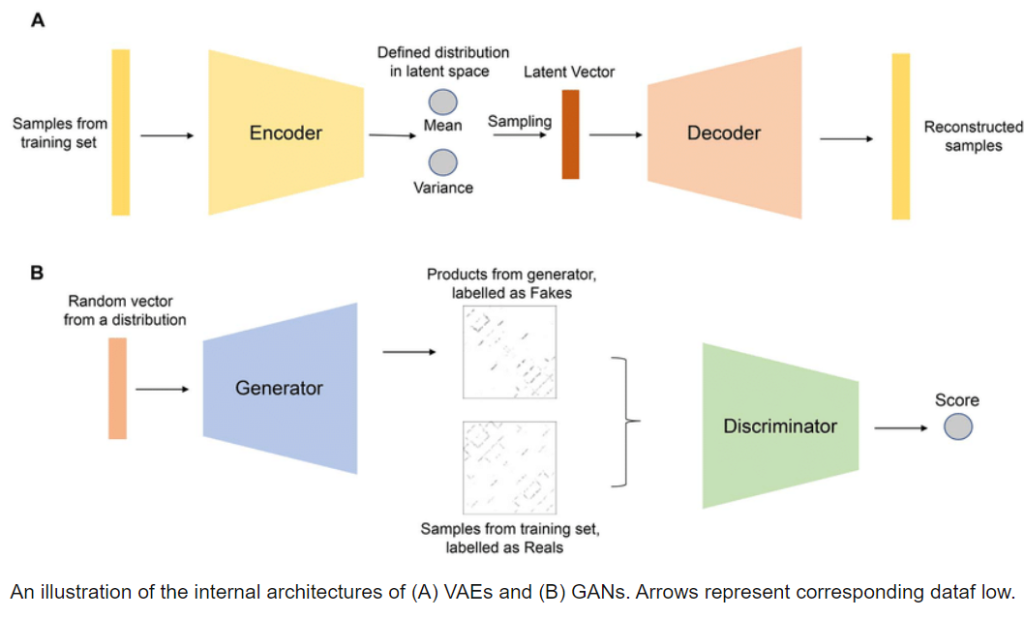
Deep learning architectures: GANs and VAEs
Generative Adversarial Networks (GANs) and Variational Autoencoders (VAEs) are two commonly used deep learning architectures for generative AI. This section explores the workings of GANs and VAEs, their differences, and their specific applications in generating synthetic data for cybersecurity purposes. Understanding these architectures is crucial to grasp the mechanisms behind generative AI models and their potential in addressing cybersecurity challenges.
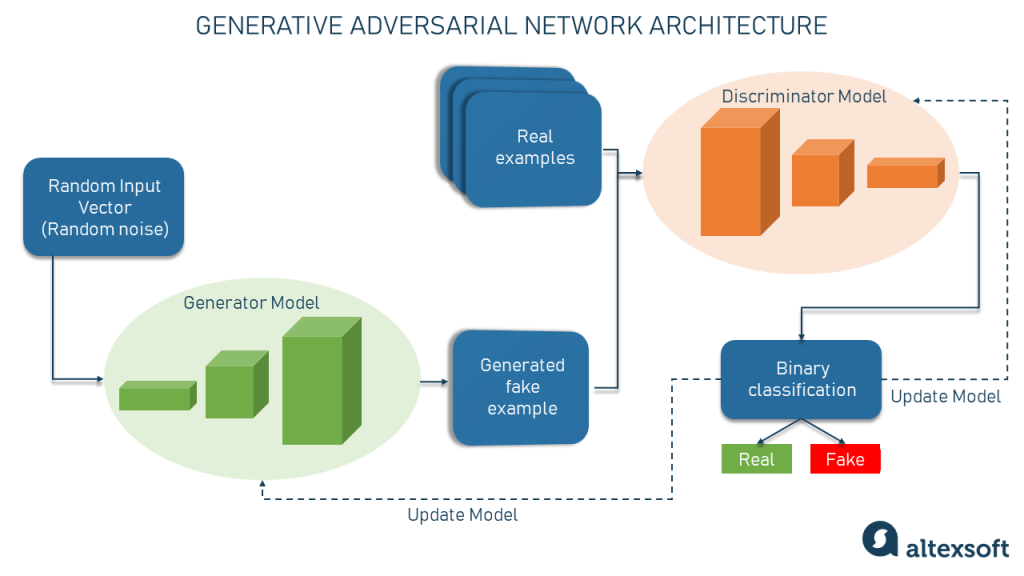
Training and generation process of generative AI models
This section explains the training process of generative AI models, including the acquisition and preprocessing of training data, model architecture selection, and the optimization process. Additionally, it explores the generation process of new data samples based on the learned patterns and distributions. Understanding how generative AI models are trained and how they generate synthetic data is vital for comprehending their practical applications in cybersecurity.
Enhancing Threat Detection and Response
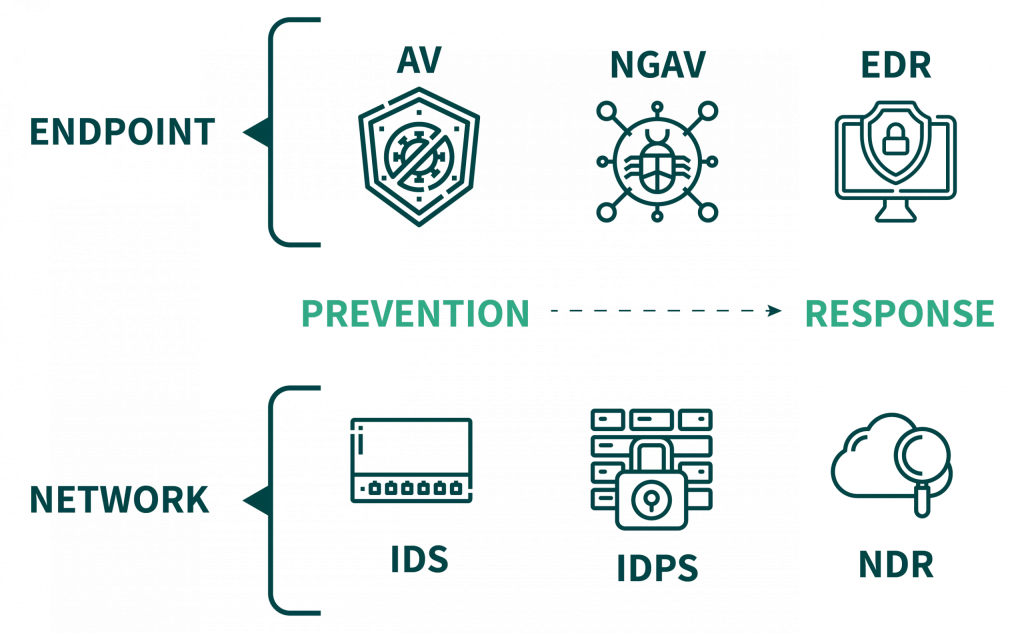
Limitations of traditional signature-based approaches: Traditional signature-based approaches to threat detection rely on pre-existing patterns and known indicators of compromise. However, these approaches have limitations in keeping up with the evolving threat landscape, as they struggle to detect novel attack techniques and zero-day vulnerabilities. This section discusses the challenges associated with traditional approaches and the need for more adaptive solutions.
Leveraging generative AI for improved threat detection: Generative AI models can analyze vast amounts of data, learn underlying patterns of cyber attacks, and generate synthetic samples that resemble new, previously unseen threats. This section delves into how generative AI can enhance threat detection capabilities by training cybersecurity systems on these synthetic samples. It discusses the potential of generative AI to detect emerging threats and improve the overall efficiency of cybersecurity defenses.
Synthetic sample generation and training cybersecurity systems: Exploring the process of generating synthetic samples for training cybersecurity systems, this section outlines how generative AI models can contribute to the creation of diverse and realistic datasets. By training on these synthetic samples, cybersecurity systems can better adapt to emerging threats and improve their accuracy in detecting and responding to attacks.
Realistic decoy systems and honeypots for diverting attackers: Generative AI can be utilized to create realistic decoy systems and honeypots that divert attackers’ attention from actual targets. This section explores the concept of decoy systems and honeypots and how generative AI can enhance their effectiveness. By analyzing the tactics used by attackers in response to these decoy systems, organizations can gain valuable insights to strengthen their defenses.
Simulating Vulnerability Assessments and Penetration Testing :
Importance of vulnerability assessments and penetration testing: Vulnerability assessments and penetration testing are vital components of a comprehensive cybersecurity strategy. This section discusses the significance of these activities in identifying weaknesses in systems and networks, as well as in evaluating the effectiveness of existing defense mechanisms.
Role of generative AI in simulating attack scenarios: Generative AI can play a crucial role in simulating attack scenarios, allowing organizations to identify potential vulnerabilities and test the effectiveness of their defenses. This section explores how generative AI models can generate synthetic attack scenarios, simulating the behavior of real-world attackers and helping organizations uncover and mitigate vulnerabilities proactively.
Identifying vulnerabilities and testing defense effectiveness: Building upon the previous section, this section explains how generative AI-generated attack scenarios can help organizations identify vulnerabilities and assess the effectiveness of their defenses. By analyzing the response to these synthetic attacks, organizations can improve their cybersecurity posture and fortify their systems against potential threats.
Proactive measures based on generated synthetic attacks: This section discusses how generative AI-generated synthetic attacks can aid organizations in taking proactive measures to strengthen their cybersecurity defenses. By understanding the potential attack vectors and vulnerabilities, organizations can implement appropriate security measures and address weaknesses before malicious actors exploit them.
Simulating Vulnerability Assessments and Penetration Testing
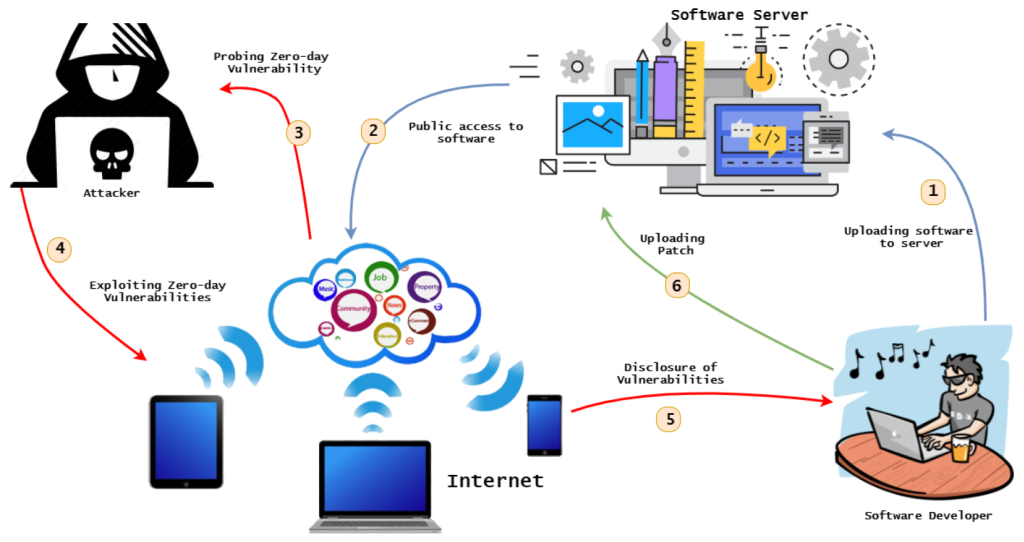
Importance of vulnerability assessments and penetration testing: Vulnerability assessments and penetration testing are vital components of a comprehensive cybersecurity strategy. This section discusses the significance of these activities in identifying weaknesses in systems and networks, as well as in evaluating the effectiveness of existing defense mechanisms.
Role of generative AI in simulating attack scenarios: Generative AI can play a crucial role in simulating attack scenarios, allowing organizations to identify potential vulnerabilities and test the effectiveness of their defenses. This section explores how generative AI models can generate synthetic attack scenarios, simulating the behavior of real-world attackers and helping organizations uncover and mitigate vulnerabilities proactively.
Identifying vulnerabilities and testing defense effectiveness: Building upon the previous section, this section explains how generative AI-generated attack scenarios can help organizations identify vulnerabilities and assess the effectiveness of their defenses. By analyzing the response to these synthetic attacks, organizations can improve their cybersecurity posture and fortify their systems against potential threats.
Proactive measures based on generated synthetic attacks: This section discusses how generative AI-generated synthetic attacks can aid organizations in taking proactive measures to strengthen their cybersecurity defenses. By understanding the potential attack vectors and vulnerabilities, organizations can implement appropriate security measures and address weaknesses before malicious actors exploit them.
Ethical Considerations in Generative AI for Cybersecurity
Responsible use of generative AI technology: As with any emerging technology, the use of generative AI in cybersecurity must be approached responsibly. This section explores the ethical considerations surrounding generative AI, emphasizing the need for developers and organizations to prioritize ethical standards and the responsible use of technology.
Addressing unintended consequences and risks: Generative AI technology brings both benefits and potential risks. This section discusses the importance of addressing unintended consequences and risks associated with generative AI in cybersecurity. It highlights the need for comprehensive risk assessments, safeguards, and ongoing monitoring to mitigate potential harm.
Privacy concerns with synthetic data generation: Generative AI models can generate synthetic data that closely resembles real individuals, raising privacy concerns. This section delves into the privacy implications of generative AI in cybersecurity and emphasizes the importance of protecting users’ privacy and ensuring compliance with relevant regulations.
Ensuring ethical standards in development and deployment: To ensure ethical standards in the development and deployment of generative AI-based cybersecurity solutions, this section discusses the importance of transparency, accountability, and robust governance frameworks. It highlights the need for interdisciplinary collaboration, involving experts from various domains, to address ethical challenges effectively.
Leveraging Generative AI in Network Security
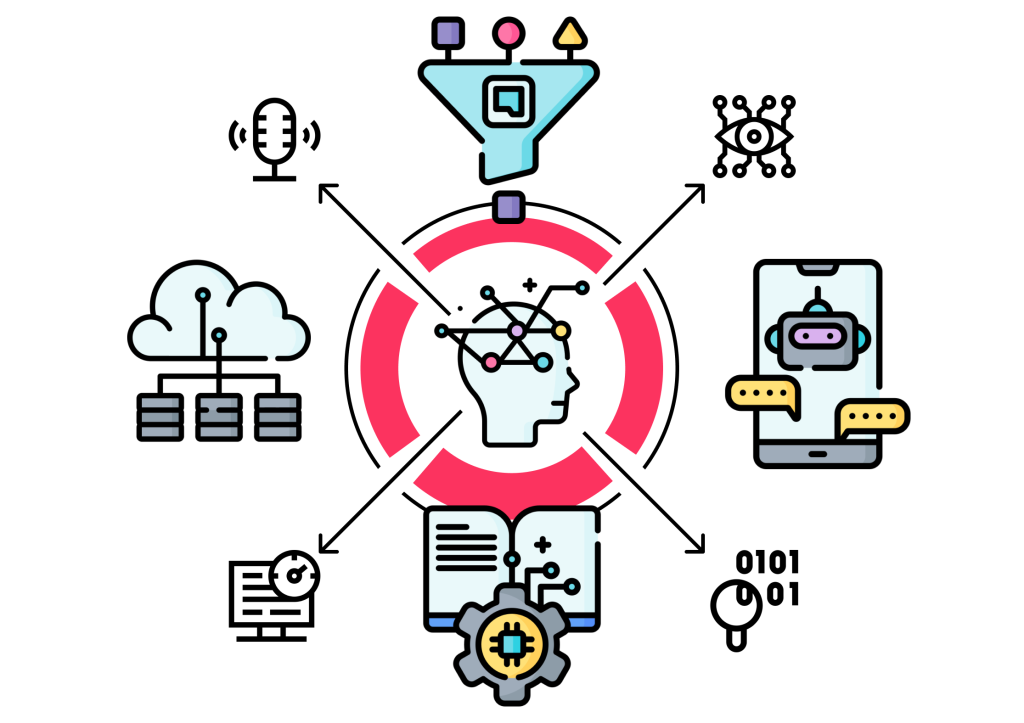
Network security challenges and the need for advanced solutions: Network security is a critical aspect of cybersecurity, and organizations face numerous challenges in securing their network infrastructure. This section provides an overview of network security challenges, including the complexity of networks, the proliferation of connected devices, and the increasing sophistication of cyber attacks.
Generative AI for anomaly detection and intrusion prevention: This section explores the potential of generative AI in network security, focusing on its applications in anomaly detection and intrusion prevention. By analyzing network traffic and patterns, generative AI models can detect abnormal behavior and identify potential intrusions in real-time.
Augmenting network security infrastructure with generative AI: Generative AI can be leveraged to enhance network security infrastructure, augmenting existing security measures. This section discusses how generative AI models can be integrated into network security systems, such as firewalls and intrusion detection systems, to improve their efficiency and accuracy.
Balancing security and performance in network environments: Network security solutions must strike a balance between ensuring robust security measures and maintaining optimal network performance. This section explores the challenges associated with achieving this balance and discusses how generative AI can help in optimizing security measures without compromising network performance.
Generative AI for Malware Detection and Analysis
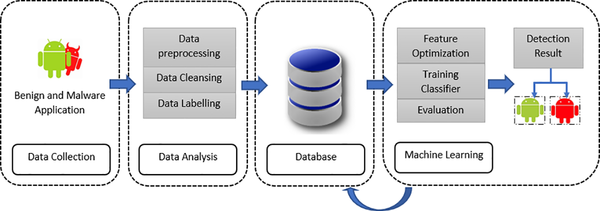
The evolving nature of malware and its impact: Malware remains a significant threat to organizations and individuals, with attackers constantly evolving their techniques to evade detection. This section highlights the impact of malware and the challenges associated with its detection and analysis.
Detecting and analyzing malware with generative AI models: Generative AI can significantly contribute to malware detection and analysis. This section explains how generative AI models can be trained to recognize malware patterns and behaviors, allowing for improved detection rates and more effective malware analysis.
Generating synthetic malware samples for training and analysis: Generative AI models can generate synthetic malware samples, enabling cybersecurity professionals to train their systems and analyze new strains of malware in a controlled environment. This section explores the process of generating synthetic malware samples and their potential applications in training and analysis.
Improving malware detection rates and response capabilities: By leveraging generative AI for malware detection, organizations can enhance their capabilities to detect and respond to new and evolving malware threats. This section discusses the benefits of generative AI in improving malware detection rates and minimizing the time between detection and response.
Challenges and Future Directions
Current challenges in implementing generative AI for cybersecurity: Generative AI in cybersecurity still faces several challenges, including the availability of quality training data, scalability, and adversarial attacks. This section explores the current challenges in implementing generative AI for cybersecurity and discusses possible approaches to address them.
Advancements in generative AI and its impact on cybersecurity: Generative AI is a rapidly evolving field, with ongoing advancements in techniques and models. This section discusses the potential impact of these advancements on the field of cybersecurity and explores how emerging technologies can further enhance cybersecurity defenses.
Collaboration and research opportunities for better solutions: Given the complexity of cybersecurity challenges, collaboration and research are crucial for advancing generative AI in cybersecurity. This section highlights the importance of interdisciplinary collaboration, knowledge sharing, and research initiatives to drive innovation and develop more effective cybersecurity solutions.
The potential for adversarial attacks and defense strategies: Generative AI models are not immune to adversarial attacks, where malicious actors attempt to manipulate or deceive the models. This section discusses the potential risks of adversarial attacks on generative AI models in cybersecurity and explores defense strategies to mitigate these risks.
Conclusion
Recap of generative AI’s role in cybersecurity solutions: This section provides a concise recap of the key points discussed throughout the blog post, highlighting the role of generative AI in addressing emerging threats, enhancing threat detection and response, simulating vulnerability assessments and penetration testing, and training next-generation cybersecurity professionals.
The potential for secure and smart systems with generative AI: Generative AI offers immense potential for creating secure and smart cybersecurity solutions. By leveraging the capabilities of generative AI, organizations can improve their defenses, respond more effectively to emerging threats, and proactively identify vulnerabilities.
Navigating ethical considerations and ensuring responsible use: As with any powerful technology, generative AI in cybersecurity raises ethical considerations. This section emphasizes the need for responsible use, addressing unintended consequences, protecting privacy, and ensuring ethical standards in the development and deployment of generative AI-based cybersecurity solutions.
Add Comment
You must be logged in to post a comment.