Snowflake vs. Data Warehouse: Clash of Innovation
In the realm of data management, the choice concerning Snowflake and traditional data warehouses has become a pivotal decision for businesses aiming to optimize their data infrastructure. With data volumes skyrocketing and the demand for real-time analytics surging, organizations seek solutions that offer flexibility, scalability, and efficiency. In this in-depth exploration, we delve into the intricacies of Snowflake and traditional data warehouses, examining their key differences, strengths, and considerations for adoption.
Understanding Traditional Data Warehouses
For many years, data warehouses have been the main engine driving corporate decision-making related to data storage, organization, and analysis. Because of this, these systems have become the foundations of enterprise data management. Warehouses of this type usually employ relational database management systems for instance: Oracle, SQL Server, or Teradata and they can be recognized by their strict data modeling which means that data is stored in tables with predefined relationships and accessed using SQL query language construction.
Strengths
Maturity and
Familiarity
One of the longstanding data management platforms that has served many companies with success is the data warehouse. It can be seen as a mature product that has already proven its value across many industries. Their proven strengths which include a wide customer base and nurturing of a community for employees and software and hardware developers make them the first on the list of organizations that can easily connect with the decisions being made.
Strong
Consistency
It is worth stressing that the nature of traditional data warehouses is heavily based on strict schema design to ensure the reliability of both data and data quality. The fact that these data lakes limit you to predefined structures and relationships makes it stay with only accurate and reliable data paving the way for the same to be applied to such use cases where precision is required such as financial reporting.
OLAP
Workloads
Classical data warehouses are designed to be suitable for carrying out complex analysis and reporting operations, which are OLAP workloads. Being based on the specialized architectures and features designed explicitly for OLAP operations, there is increased robustness in terms of performance, interactions, and storage management for processing huge data volumes and assistance in insights generation.
Challenges
- Scalability Limitations- With their clear advantages, still apps might stumble on stretching to fit the ever-growing mass of data generated by enterprises. Since the expansion of these warehouses generally needs considerable spending upfront for equipment and infrastructure, mainly achieving proper planning and resource allocation, is the key to success. Traditional warehouses often come with the bagpipes of scalability limitations that can be a pain in the neck for organizations that desire to leverage the full power of their data for business flexibility and not only growth but also survival through evolution.
- Limited Flexibility- Static data warehouses could face difficulties in agility and flexibility, especially while environments are changing very fast and data schemas experience frequent changes. Such an approach could block the possible flexibility that organizations need for rapid reactions to the changing business landscape and the inclusion of non-traditional sources of data. The reduction in effectuality due to the nature of the conventional warehouses precludes the organization from creating new ideas and discovering new knowledge from mixed datasets.
- Cost and Complexity- Keeping and maintaining traditional data warehouses may be an expensive and difficult issue that wants for these database systems to have a variety of specially trained and needed skills and resources administration, optimization, and maintenance. From device procurement and configuration to country licensing and ongoing support, the entire circle of traditional warehouse maintenance processes becomes ‘cumbersome’, which threatens to intensify the expenditure and overburden organizational resources. Moreover, the write-up of data is getting involved as the data volumes and usage rise which demand the use of the proper cost management tips and resource optimization strategies.
Established data warehouses are dedicated to maturity, familiarity, and solid integration while they can have drawbacks with regards to expanding capacity, flexibility, and price. Organizations have to perform the crucial yet complex task of weighing the pros and cons of utilizing traditional data warehousing against the newest information technology tools, such as Snowflake. The most appropriate solution for your information needs will ultimately be determined by the evaluation process.
Snowflake
Snowflake is a tech-driven revolution when it comes down to data warehousing. In essence, it debuts scrambling the way companies stack, administer, and analyze their data. Snowflake, has been built with a cloud-native architecture which enables the platform to employ a suite of capabilities and functionalities to overcome the issues faced by traditional data warehouses.
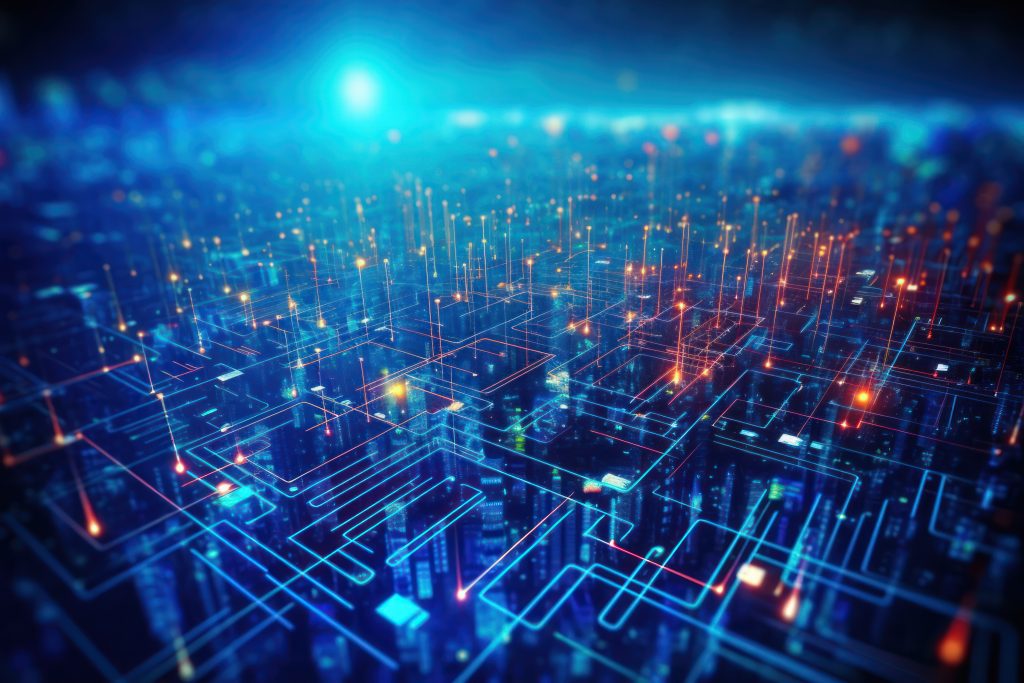
Strengths
- Elastic Scalability- At the heart of Snowflake’s architecture is an elastic scalability property, the feature defining Snowflake’s main strength, and enabling organizations to expand or reduce computing resources(simply by scaling up or down) alongside unpredictable workload fluctuations. This is a rather significant contrast to conventional warehouses which in most cases would have to employ manual management through the employment of a staff and also provide hardware that is capable of supporting an increase in storage, which results in rapid and uninterrupted growth. This flexibility allows the organizations to process peak workloads, unite sudden data volumetric growth, and decrease loading in the case of excessive inactivity with no build-up in performance levels.
- Separation of Storage and Compute- The innovation flaw of Snowflake is the fact that it takes up a different approach opposing the traditional data warehouse where storage and computing are pulled together as one resource. This enables to avoid extra allocation of resources and instead be ready to do dynamic resource allocation by each organization’s specific needs. Since in Snowflake independent power of storage and compute resources are separable, the utilization of resources is maximized optimally and costs are reduced along with this which results in efficiency. This pay-as-you-go model not only makes operations more straightforward without the added overhead but also ensures that organizations only pay for what they use, thus, leading to a reduction in variable costs over the long run.
- Semi-Structured and Unstructured Data Support– As opposed to traditional storage, which can be set up for keeping structured data only, Snowflake provides built-in options for various types of input files including JSON, Avro, and Parquet. This native support functions as an accelerator to organizations’ data ingestion, storage, and analysis tasks, which frees recognizable types and schema requirements. It does not matter from which place this data is coming – from edge-to-center devices like IoT, log files, or social media feeds — Snowflake makes it possible to generate highly valuable insights from a wide variety of data sources thus giving the end users a clear, comprehensive, and an appropriate picture to work with. The removal of the data preparation hassles in Snowflake supports the efficient data pipeline management that, in turn, reduces time-to-insight and eventually leads organizations to make faster informed data-driven decisions.
Challenges
- Learning Curve- Although Snowflake is Marveled by its capabilities to scale up and integrate with versatile systems, such organizations will likely have to experience a certain learning curve, especially those familiar only with mainstream data warehousing concepts and approaches. Considering the training and upskilling as necessary initiatives to guarantee the data professionals obtain the right skills to operate data correctly with the help of the Snowflake platform. This situation, though, is not a hindrance forever. Numerous resources, documentation, as well as community assistance, are just a few of the sources that can help grow over this initial struggle and allow full utilization of why Snowflake stands out.
- Vendor Lock in- Since Snowflake is a cloud-based solution, it might create an issue of locking companies into the provider and later they cannot make changes to their data infrastructure. Besides that, Snowflake is flexible and can run at scale. However, it is much better for enterprises to also think about the provider risks including the use of a single provider, data disruptions, and portability impairments. To reduce these risks, organizations need to design robust exit strategies, evaluate alternative solutions, and give data portability high priority as part of their data architecture design.
- Cost Management- While Snowflake’s free pricing model carries with it the advantage of cost savings and flexibility, however, user governance should be in place to make necessary changes and avoid charges that are beyond our budget. Meticulous workload management, resource allocation and performance tuning are the most significant means to deepen the effectiveness and help the organization gain the maximum possible value from its Snowflake investment. Through means of real-time usage pattern monitoring, the identification of optimization opportunities, and the deployment of effective cost management practices, organizations will be able to get the best of what snowflake solutions offer in terms of scalability and flexibility without losing control over their cloud costs.
The Snowflake data warehouse stands out by its unique ability to scale up on demand and provide a high degree of flexibility in terms of data management while being far more efficient than the other warehouses. Organizations would leverage the cloud-native Snowflake architecture and its innovative features to maximize new data-based innovation possibilities, speed time-to-insight, and, more importantly, improve their competitive position in the ever-changing market. Yet, it is extremely significant to overcome these obstacles while covering the sphere of both technical and price policies, therefore, in no way Snowflake can stop evolving as the strategic tool delivering data management and analytics services.
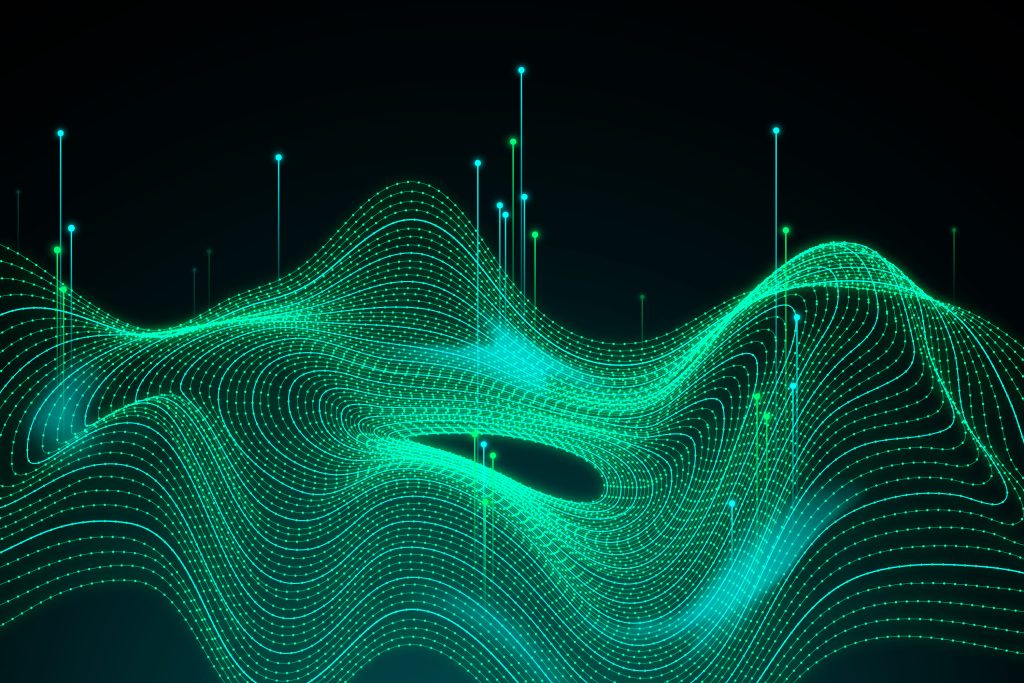
To sum it up
In the evolving landscape of data management, the choice between Snowflake and traditional data warehouses is not merely a matter of preference but a strategic decision that can significantly impact an organization’s ability to harness the power of data. While traditional data warehouses offer familiarity and consistency, Snowflake introduces a new era of scalability, flexibility, and efficiency. By carefully evaluating the strengths, challenges, and unique requirements of their data ecosystem, organizations can navigate the data landscape with confidence and choose the solution that best aligns with their goals and objectives.
Here is the link to my previous blog- SharePoint: 5 Ways to Supercharge Your Business